In the field of chemical engineering, the issue of ethylene epoxidation is akin to the famous Goldbach Conjecture in the field of mathematics, recognized as a long-standing and challenging problem within its domain.
Ethylene epoxidation is one of the significant reactions in the ethylene chemical industry, with a development history of approximately 50 years. Currently, the international industry has achieved a production scale reaching tens of millions of tons.
Despite years of theoretical research and experimental experience in this direction, there is still no unified consensus on the reaction mechanism of ethylene epoxidation within the field, and many fundamental physical and chemical issues remain controversial.
For instance, in silver catalysts, is the catalytic site more active as silver oxide or metallic silver?
The reasons for this include, on one hand, the main issue in theoretical research is that researchers can only conduct calculations based on the principles of quantum mechanics. After some structures are decomposed, they become "seemingly correct but actually incorrect."On the other hand, in experimental science, the surface structure of silver catalysts is usually produced under high temperature and high pressure conditions, and scientists usually use offline detection methods.
Advertisement
However, this method can only obtain relatively vague vibrational spectroscopy information, and it is difficult to detect active sites under such reaction conditions, so the specific mechanism of the reaction process is not very clear.
Based on this, Professor Liu Zhi Pan's team from Fudan University used machine learning atomic simulation to reveal the active sites of silver catalysis for ethylene epoxidation.
In this study, the reason why the silver (Ag) (100) surface is selective for ethylene epoxidation was determined: there are five sub-surface coordinated oxygen atoms (O5) in the complex reconstruction of the Ag (100) surface formed under reaction conditions.
This study not only carried out innovative theoretical calculations, but also proved them with experiments, providing an answer to the long-term debate on the active sites of silver catalysis for ethylene epoxidation in the field, which is conducive to promoting researchers to design and control catalysts at the atomic level in the future.The reviewer commented that the study is a perfect combination of first-principles calculations assisted by machine learning and statistical mechanics calculations. Another reviewer believes that the paper is technically very advanced, comprehensive, and systematic, and the results have very important industrial significance.
Recently, the related paper was published in Nature Catalysis with the title "Square-pyramidal Subsurface Oxygen [Ag4OAg] Drives Selective Ethene Epoxidation on Silver" [1].
Fudan University doctoral students Chen Dongxiao and Chen Lin are the first authors, and Professor Liu Zhi Pan is the corresponding author.
Piercing the "window paper" of technology, achieving 500,000 structural space sampling within one week.The complexity of chemical reactions lies in the unknowns of the reaction mechanism, the pathways of the reaction, and the surface structure.
In this study, machine learning atomic simulations can truly delve into the atomic level of catalysis, which is inseparable from the "first tool"—machine learning.
However, the problem with general machine learning potential functions is that there is no way to perform sufficiently complex potential energy surface sampling, hence the predictive capability is relatively limited.
In 2022, with the development of AI technology and the team's years of technical accumulation, they realized that the time had come to break through the "window paper" of exploring the active sites of silver-catalyzed ethylene epoxidation.
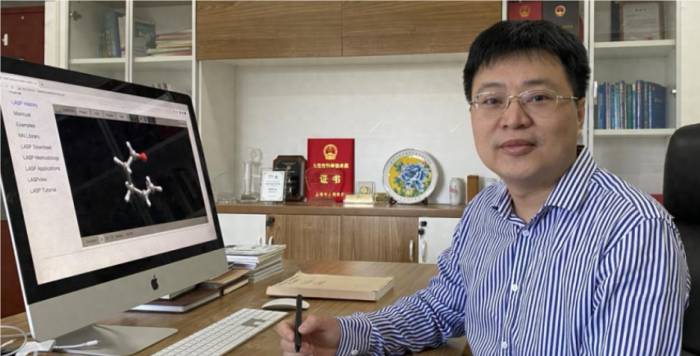
This research took about two years, and the researchers, based on machine learning methods, theoretically demonstrated a "digital twin" of the surface structure of silver catalysts.For the first time, a clear representational image has been revealed, including the reconstruction of the silver catalyst surface from Ag(100), Ag(111), and Ag(110) under reaction conditions, to the epoxidation of ethylene.
Liu ZhiPan said: "Through machine learning atomic simulation, we can provide all the possibilities and all the data at the bottom level. With these data, we can combine quantum mechanics calculations, micro-kinetic simulations, and online experimental equipment to clarify the historical issues of the ethylene epoxidation mechanism."
After screening the structures of different configurations of the Ag surface, the researchers confirmed the optimal surface structures of three Ag surfaces under the conditions of 500K, 1bar ethylene, and 1bar oxygen. Under these conditions, a total of four coexisting phases were determined.
Different from the other three coexisting phases that only have surface oxygen, in Ag(100), the unique surface oxide phase O5, in addition to having surface oxygen, also includes subsurface oxygen with five coordination [Ag4OAg], as well as strongly adsorbed ethylene with special electronic properties.
Subsequently, the researchers conducted in-depth research on the reaction mechanism of ethylene on the above surfaces by using the self-developed reaction path automatic sampling algorithm.The results show that only the O5 phase can promote the formation of ethylene oxide; while in other phases, the combustion reaction is first carried out to carbon dioxide, which adversely affects the selectivity of ethylene oxide.
The research is mainly divided into three stages, including the evaluation and experimental exploration of potential functions, time cost, and computational efficiency.
Firstly, the potential function. The potential function can comprehensively and accurately describe the interactions between silver/silver oxide and carbon-oxygen-hydrogen organic compounds (such as ethylene, ethylene oxide, propane, etc.).
It is understood that the research group has previously obtained the potential function of silver-carbon-hydrogen, and the researchers' goal is to optimize it to 3-4 milli-electron volts per atom. Only when the accuracy is high enough can unknown structures, transition states, reaction rates, etc., be predicted through this parameter.
Secondly, the evaluation of time cost and computational efficiency. In this research, the researchers have achieved the completion of about 500,000 structural space samplings within one week.Thirdly, experimental detection. After completing the theoretical research, researchers concluded that the ethylene adsorption on the phosphorus surface caused the carbon-carbon double bond vibration peak to reach 1583 cm^-1 after in-situ infrared spectroscopy characterization.
Liu Zhi Pan recalled and said: "What is exciting is that this stable structure has never been seen in the field of chemistry. Moreover, the experiment corresponds to the theoretical infrared simulation results of ethylene in the O5 phase, confirming the accuracy of the theoretical prediction of the active site in the O5 phase."
Let catalytic theoretical calculations "run" before experiments
This study, for the first time, used machine learning methods to reveal the catalytic properties of silver under ethylene epoxidation reaction conditions through calculations, opening up the space for imagination in the field of catalysis research."In principle, any catalytic reaction, the process of a chemical reaction, can be determined for its surface structure under the reaction conditions using this method, following the same workflow. Subsequently, one could also attempt to replace silver with gold, perovskites, etc., to verify and discover other epoxide reactions," said Liu Zhi Pan.
In the field of science, many seemingly complex problems are actually quite simple in essence.
Liu Zhi Pan pointed out that before the development of this new technology, looking at problems was similar to "a blind man feeling an elephant," but with this method and technology that is good enough to use, it is equivalent to looking at problems from a global perspective, so the problems also become relatively simple.
He believes that the future trend of scientific research development will be driven and led by theoretical calculations. On one hand, this approach is cost-effective; on the other hand, it can also save the manpower cost of conducting experiments.
"Theoretical calculation-driven methods will change our scientific research paradigm. Based on this, all aspects of scientific research, including the laboratory atmosphere, laboratory structure, and the knowledge reserves required by doctoral supervisors, will undergo changes."In addition, in the field of chemical reactions and the design of catalysts, Liu ZhiPan believes that within the next 10 years, a new type of catalyst may emerge that is led by theory and then recognized and discovered from an experimental perspective.
Building a Unique Scientific Research Ecosystem with Original Innovation
Liu ZhiPan graduated from the Chemistry Department of Shanghai Jiao Tong University with a bachelor's and master's degree, then obtained a Ph.D. in Theoretical Chemistry from Queen's University Belfast in the UK, and engaged in postdoctoral research at the University of Cambridge.
Starting from August 2005, he has established an independent research group in the Chemistry Department of Fudan University and serves as a professor and doctoral supervisor. Currently, the main research direction of Liu ZhiPan's research group is to develop new theoretical calculation methods for catalytic mechanism research and material design to guide catalytic experiments."When I first returned to the country to teach, computers were not only expensive but also had very limited computing power. Therefore, at that time, the basic field was mainly based on first-principles calculations to study known systems and electronic structures," Liu Zhi Pan recalled.
He has always believed that in the field of scientific research, China needs to establish its own original innovation, thereby leading the development of science through the invention of innovative technologies.
In recent years, Liu Zhi Pan's research team has gradually built a unique ecosystem through a series of theoretical methods, from exploring potential energy surfaces to establishing potential functions, and then to various surface structures or micro-dynamics combined with reaction pathways.
In 2013, he led the team to develop a global optimization method called Stochastic Surface Walking (SSW), which made it simple and feasible to find key structures on potential energy surfaces.
However, SSW is limited by the slow speed of the method and can only perform simple chemical reactions involving about 10 atoms.To address this issue, Liu Zhi Pan and his team members have conducted a three-year exploration in the direction of solid phase transitions, starting from scratch by writing their own code and exploring neural networks, until they developed a potential function, and published a related paper in 2017 [2].
"This research is a significant breakthrough for our research group. We are confident that we can predict the complex and high-dimensional material space, and we hope to use this method for atomic simulation in the future," said Liu Zhi Pan.
In 2018, they independently developed the Large-scale Atomic Simulation with neural network Potential (LASP) platform for predicting structures and reaction pathways, which has been updated to version 3.6.0 to date.
LASP is not only a research tool within the research group but also open to all researchers through the website ().
It is reported that in 2023, the research group of the University of Science and Technology of China constructed a new type of artificial carbon crystal C60. Before the experiment, it was based on LASP theoretical simulation calculations for related experiments, and the related paper was published in Nature [3].Recently, by integrating GPU computing, the research team has developed a potential function for the exploration of the entire lifecycle, and has achieved a very high level of computational resources.
Based on this, Zhi-Pan Liu believes that in the future, it may even be possible to skip the step of potential functions and start researching directly from material design and reaction design, which will greatly accelerate the discovery and development of the field.
Compared with predicting the real world using fundamental laws, AI has the advantages of low cost, fast speed, and high efficiency by learning and discovering scientific laws through big data.
Recently, the team is also promoting some horizontal projects, such as enterprises hoping to predict key data in the field of semiconductors, including atomic images, thermodynamics, dynamics, etc., through leading theoretical calculations combined with AI.
"In the future, in fields such as material design and biomedicine, it is expected that AI will lead the development of projects. We will also continue to deepen the direction of the combination of AI and catalysis, chemistry, and hope to make more contributions to the field," said Zhi-Pan Liu in conclusion.