In recent years, the development of large language models has been unstoppable. However, they still perform poorly in tasks that require understanding implicit instructions and applying common sense knowledge.
For example, multiple attempts are needed to achieve human-level performance, which leads to inaccurate responses or inferences of large language models in real-world environments.
To address the above issues, recently, a research team from Kunming University of Science and Technology proposed an "Internal Time-Consciousness Machine" (ITCM) based on the computational structure of consciousness, and built an intelligent agent (ITCMA) based on ITCM.
This intelligent agent supports behavior generation and reasoning in an open world, enhancing the ability of large language models to understand implicit instructions and use common sense.
The research group introduced the Alfworld environment to test the ITCMA and found that the trained ITCMA performed 9% higher on the dataset it had seen than the current best level; while the untrained ITCMA also achieved a task completion rate of 96% on the dataset it had seen, which is 5% higher than the current best level.This result indicates that the intelligent agent has surpassed traditional agents in terms of practicality and generalization ability.
Advertisement
It is worth mentioning that the team also deployed ITCMA to a quadruped robot to carry out experiments on its effectiveness in the real world. (Specific results can be seen at )
The results show that the untrained ITCMA has a task completion rate of 85%, which is close to its performance on unseen datasets, proving that the intelligent agent is practical in real environments.
From an application perspective, this intelligent agent is expected to have a good prospect in the following areas.
Firstly, in the field of companion robots.Currently, some studies have demonstrated that people can develop attachment projections even towards relatively simple digital humans.
Thus, digital humans based on generative agents could elicit stronger attachment projections from people. In this case, the agent may play a significant role in the field of companion robots.
Secondly, in the field of virtual psychological counseling.
In fact, due to the presence of issues such as empathy and ethics, the psychological counseling industry has always been cautious about AI-provided psychological counseling and interventions in the past.
After the emergence of large language models, the industry found that AI can at least help solve some simple psychological intervention problems, thereby alleviating to some extent the pressure on the industry caused by the shortage of mental health professionals.So, the digital human based on ITCMA, which includes emotions and mirroring, may help to solve the empathy problem that existing large language models face in deep interaction with humans.
Recently, the relevant paper was published on the preprint platform arXiv with the title "ITCMA: A Generative Agent Based on a Computational Consciousness Structure" [1].
Zhang Hanzhong, a master's student at Kunming University of Science and Technology, is the first author, and Associate Professor Yin Jibin of Kunming University of Science and Technology is the corresponding author.
According to Zhang Hanzhong, the research began in 2021.
At that time, the academic community generally believed that general artificial intelligence, that is, strong artificial intelligence, is still quite far from human beings.So, most researchers in this field choose to focus more on weak artificial intelligence used to complete a specific task.
Neural networks are generally considered a part of weak artificial intelligence. As the development of this "black box" algorithm continues to deepen, the academic and industrial communities are also paying more and more attention to its interpretability.
Not only that, but the effectiveness of neural networks will also be greatly reduced after the problem domain changes.
It is based on the above background that the research group chooses to take artificial psychology as the direction of this research.
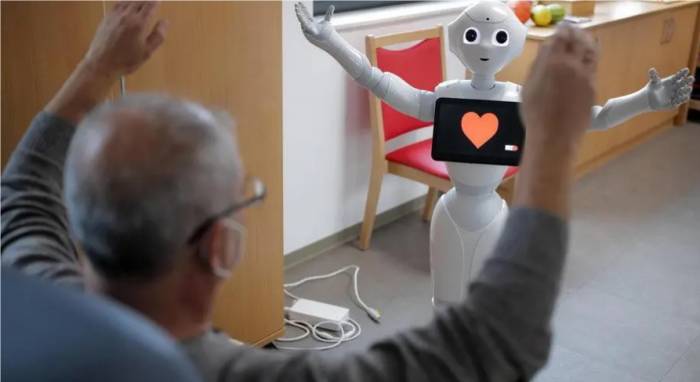
However, as the research gradually deepens, their research direction has also slowly become an interdisciplinary study centered on human-computer interaction, including cross-disciplinary psychology, sociology, philosophy and other disciplines.In 2022, the birth of ChatGPT demonstrated that models with a sufficiently large parameter scale have the potential to exhibit emergent capabilities.
Furthermore, a team from Stanford University in the United States, with a large language model at its core, proposed a generative intelligent agent. This indicates that the intelligence emerging from large language models can possess a certain degree of sociability.
For a generative intelligent agent, its input object is the current environment and past experiences, while the output object is the generated behavior.
The foundation for generating this behavior comes from a novel intelligent agent architecture that can combine large language models with mechanisms for synthesizing and retrieving information, providing conditions for the output of the large language model.
Without the aforementioned mechanisms, although the large language model can still output behavior, the resulting intelligent agent may not be able to respond based on past experiences, make important judgments, and thus fail to maintain long-term consistency.Here is the English translation of the provided text:
For example, in the virtual environment project "Stanford AI Town" developed by researchers from Stanford University and Google, multiple agents are placed in a small town and allowed to interact freely without any intervention.
In this process, the role of the agents is to make the non-player controlled characters in the town respond according to the different actions of the players.
The birth of this research has also driven a large number of agent-related results to emerge in 2023. Most of them are based on a connectionist idea, believing that the success of large language models can allow agents to be reduced to the results produced by complex systems.
"In fact, we do not fully agree with the above idea. Even though GPT-4 and Claude 3 have surpassed humans in many aspects, many issues still exist, including memory and hallucinations.
Therefore, generative agents using large language models as the 'brain' also cannot avoid having these limitations," said Zhang Hanzhong.
Note: The translation is provided in a clear and accurate manner, ensuring that all the key information from the original text is conveyed in English.From a disciplinary perspective, cognitive neuroscience differs from the neuroscience that forms the underlying foundation of the brain in biology.
The former is less concerned with which neurons there are and how they are connected, but rather more focused on the "brain regions" as the upper-level structure, which are the functional parts of the brain that are interrelated yet each perform their own functions.
This research group believes that research on general artificial intelligence is similar.
Therefore, in this case, instead of focusing on how the underlying neural networks are constructed, it is better to deconstruct the entire "brain" based on existing research, using a computational consciousness structure capable of imitating human consciousness as the basic theoretical model, to handle some simple rule sets such as consciousness flow, memory, emotions, etc.
"At that time, we believed that weak artificial intelligence, which is solely based on neural networks, is difficult to develop into general artificial intelligence.Therefore, under the influence of models such as integrated information theory, the Turing machine of consciousness, and combining the phenomenological model of consciousness persistence from psychology and philosophy, we have proposed the ITCM structure, said Zhang Hanzhong.
Based on the ITCM structure, the team constructed the generative intelligent agent ITCMA, aiming to provide a framework for behaviors in an open world that can interact with other intelligent agents and respond to environmental changes.
That is to say, by computationally decomposing consciousness through ITCM, researchers can obtain an intelligent agent with "consciousness," even if this consciousness is a rather rudimentary one.
"ITCMA can both 'actively' do something through its own ideas and complete the transfer of experience across tasks through the similarity of the phenomenological field in ITCM, and even learn how to complete tasks autonomously without any experience," said Zhang Hanzhong.
They have demonstrated through experiments that ITCMA can learn how to find an item using the tools provided by the environment without any guidance, and place it in a cabinet after freezing it, all within a very short time (within 20 steps).Obviously, it is precisely through the transformation of the research perspective of the discipline that they were able to correct a series of flaws caused by the traditional generative intelligent agents' excessive dependence on large language models.
According to Zhang Hanzhong, the successful establishment of the ITCM model is also due to the help provided by a friend of his who is studying psychology.
"In discussing how to express the ITCM model, she started from a psychological perspective and gave me a lot of suggestions.
Moreover, she also established a set of psychological theories called 'Dream Theater' based on this, and published it on Douban. In the future, it may to some extent become the psychological theoretical foundation of ITCM," he said.
In fact, in the current research on ITCMA, researchers have only studied the effect and learning ability of the intelligent agent as a single intelligent agent, and have not further studied what kind of cooperation and interaction effects it can show when multiple intelligent agents exist simultaneously.So, next, they plan to focus on researching the social collaborative strategies of ITCMA in the social network environment.
"In this study, perhaps many people pay more attention to the structure and experimental results of ITCM, but I and other members of the team believe that the most important part is reflected in the models that are not aimed at committing to the task, as well as the advanced functional interpretation of ITCMA awareness," said Zhang Hanzhong.
They attach great importance to the differences between ITCMA and traditional agents, and are committed to making it have sufficient "subjectivity".
Just like the original philosophical definition of the concept of "agent", they expect generative agents to be entities that have desires, beliefs, and intentions, and can take autonomous actions.
"Proactivity is always the most important characteristic. We hope that ITCMA is not just a task-driven tool, but also has its own ideas and can do something autonomously," said Zhang Hanzhong.