The disease-resistant phenotype prediction method we have developed has an accuracy rate of over 90% for predicting rice blast, wheat stripe rust, and leaf rust diseases, indicating that AI is expected to greatly improve the efficiency of disease-resistant variety screening and provide a new method for screening disease-resistant germplasm resources and varieties. "Said Kang Houxiang, a researcher at the Institute of Plant Protection, Chinese Academy of Agricultural Sciences.
In a recent study, he and his team found that machine learning can be used to predict the disease resistance of crops and developed the above new method.
By obtaining disease resistance-associated markers through genome-wide association analysis and screening markers within an appropriate P-value range, this method can use these markers to quickly and accurately screen disease-resistant resources and varieties, saving both time and manpower.
Based on the genotype and existing machine learning methods combined with kinship, such as lightGBM_K, RFC_K, and SVC_K, and other machine learning methods, it can accurately predict rice blast, black stripe dwarf disease, and sheath blight, as well as predict the resistance level of wheat to stripe rust and leaf rust diseases.
Advertisement
In the study, the research team also provided the core rice variety's resistance identification results for multiple diseases.For breeding companies, by integrating the already mature and cost-effective whole-genome single nucleotide polymorphism (SNP) marker detection technology, it is possible to accurately determine the resistance to various diseases for all parents, intermediate varieties, or existing commercial varieties.
At the same time, by combining the parental materials of the breeding company, based on the foundation of this machine learning method, one can establish an exclusive process to achieve efficient and digital disease-resistant variety screening, reducing the cost of disease-resistant variety screening and improving screening efficiency.
In addition to disease resistance, this method can also play an auxiliary role in screening for other excellent traits.
Why is the price of field disease identification still high?
It is reported that on a global scale, current food production safety still faces some major challenges.For instance, the prevalence of major diseases such as rice blast, wheat rust, and Fusarium head blight often leads to reduced yields or even total crop failure.
Therefore, the selection and cultivation of disease-resistant varieties are of great significance for ensuring the safety of grain production.
Unlike traits such as crop yield and appearance quality, the disease resistance of crops is a trait that is difficult to measure accurately.
Thus, in the breeding process or the production and cultivation process, how to accurately screen for disease-resistant materials from tens of thousands of breeding materials, and how to accurately select disease-resistant varieties from numerous cultivated varieties, has always been a goal that plant protection scientists and breeders strive to achieve.
In current production practices, the screening of disease-resistant varieties relies on field disease identification.For some major diseases such as rice blast disease in the field resistance identification, the current market price is approximately 1000 RMB/variety/location, which is both costly and time-consuming.
For example: Suppose a breeding company generates 10,000 intermediate materials through different combinations during the breeding process, it is necessary to accurately select materials resistant to rice blast disease from these 10,000 intermediate materials.
If traditional field resistance identification methods are used, the cost is often as high as 10 million RMB, and it takes at least a production season to complete.
Research shows that the integration of AI with the industrial sector has greatly promoted the development of related industrial research and the industry, and the combination of AI and agriculture is still in its infancy.
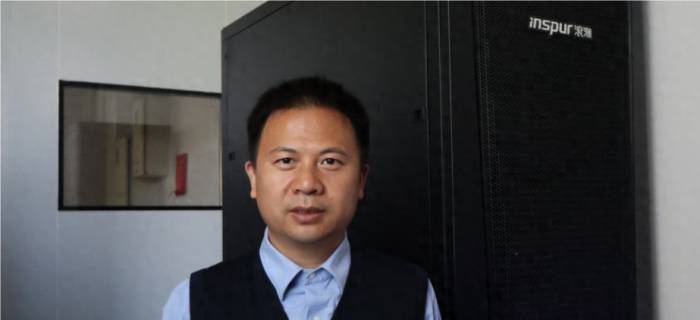
As a scientific researcher engaged in agricultural production, Kang Houxiang found that in agricultural production, there are often some problems that are difficult to solve with traditional methods.
For example, how to accurately select varieties with high yield, good quality, and strong resistance to diseases from thousands of varieties with similar appearances for the cultivation of the next generation of excellent varieties? And can it achieve the goal of "reducing the price" of traditional methods?Based on years of experience in data analysis, Kang Houxiang realized that perhaps machine learning could solve these problems.
With the successive launch of AI tools such as AlphaGo and AlphaFold, his idea of using AI to solve agricultural production problems became even more firm.
Based on this, Kang Houxiang and his colleagues began to use machine learning to improve the efficiency of screening for disease-resistant crop varieties.
He hopes to develop a new method that can accurately screen for disease-resistant varieties while significantly reducing costs, thereby improving the efficiency of disease-resistant breeding.When agricultural researchers began to self-study Python
The real decision for Kang Houxiang to embrace AI can be traced back to the end of 2019. At that time, the COVID-19 pandemic suddenly broke out, and he was confined at home for a long time without the ability to go out.
As a result, he tried to change the conventional thinking pattern that mainly relied on wet experiments and started to consider how to use machine learning methods for scientific research.
After having this idea, Kang Houxiang began to learn the computer language Python. He found that Python can not only flexibly mobilize machine learning libraries but also has many mature machine learning frameworks.
In fact, before the COVID-19 pandemic, he and his team had already built a method combining machine learning around the data accumulated in the laboratory.On this basis, he hopes to quickly and accurately predict the disease resistance of new crop varieties, breaking the traditional methods of time-consuming and labor-intensive field disease resistance identification, thereby improving the efficiency of disease-resistant breeding.
However, it is not an easy task to learn a computer language and immediately use it to solve scientific research problems.
Among agricultural researchers, there were also very few people who used Python for their projects before. Sometimes, a small syntax error in the program may take half a day to solve.
However, hard work pays off. About two months later, Kang Houxiang learned to use dense neural networks in the PyTorch framework for image recognition.
At the same time, he used one-hot encoding to solve the problem of transforming from seed genotype to seed image, learned to use neural networks for machine learning, and also learned to use machine learning methods to classify data.To handle raw data and optimize the analysis process, Kang Houxiang and his team attempted two methods.
The first method was to utilize the original SNP data; the second method was to use the Genome-wide association study (GWAS) to find SNP data associated with disease resistance.
By doing so, they discovered that compared to the first method, the second method not only took less time in the subsequent machine learning process but also had a higher accuracy rate.
After obtaining the associated SNP data through GWAS analysis, the research team carried out data testing and selected different P-value thresholds as data inputs.
The results showed that both too large or too small P-value thresholds are not conducive to establishing accurate predictive models for machine learning. Through this, they also found the optimal P-value thresholds for several important diseases.During the establishment of machine learning predictive models, in the sampling of machine learning, they adopted the method of random sampling.
Their findings showed that after establishing a phylogenetic tree based on population kinship, and then learning through uniform sampling from the phylogenetic tree, the predictive accuracy of the model can be significantly improved.
This indicates that for machine learning, models established by the method of uniformly feeding data are more accurate than those established by randomly selecting data.
Finally, they used the established machine learning model to predict rice blast disease.
The prediction results show: with the help of this model, the resistance of any new variety can be accurately predicted based on genotype alone, with an accuracy rate of over 90%.Subsequently, for rice sheath blight and rice black streak dwarf disease, two diseases in rice that are widely recognized as difficult to identify for resistance, the research team also achieved high accuracy with the help of machine learning models.
When applied to the prediction of wheat blast and stripe rust, the team also achieved high accuracy. Through individual inoculation identification, they further confirmed the authenticity of the predicted results.
Recently, the related paper was published in Engineering with the title "Development of Machine Learning Methods for Accurate Prediction of Plant Disease Resistance" [1].
Liu Qi, a graduate student at the Institute of Plant Protection, Chinese Academy of Agricultural Sciences, and Professor Zuo Shimin from Yangzhou University/Zhongshan Laboratory of Biobreeding, are the co-first authors, and Kang Houxiang serves as the corresponding author.
It is reported that Zuo Shimin has undertaken a large amount of disease resistance identification work. As mentioned earlier, rice sheath blight and rice black streak dwarf disease are two diseases in rice that are extremely difficult to identify for resistance."However, Zuo Shimin has been diligent and uncomplaining, leading the team to complete the resistance identification of rice dwarf disease in multiple locations and multiple pathogenic types of sheath blight for hundreds of rice varieties," said Kang Houxiang.
At the same time, the successful completion of this achievement also made Kang Houxiang truly realize that opportunities are only given to those who are prepared.
Before this project, he had accumulated years of data analysis experience and often self-studied code programming through online videos and purchasing books.
This allows him to combine his strengths and the trend of AI in the increasingly popular era of AI For Science, to make new achievements that fit the background of the development of the times.
Subsequently, Kang Houxiang plans to cooperate with breeding companies to strive to promote this technology to the application market.