In the digital economy era, deepfake technology, represented by "Deepfake," has become more intelligent and highly realistic. Especially with the rapid development of large models, deep fakes have become more realistic, the scenarios more complex, and the technology variants faster and more numerous. At present, deepfake technology is illegally used in economic, political, and social fields, posing a serious threat and being rated as the top of the "Top Ten Global Risks in the Next Two Years." The detection of deepfakes faces greater challenges and higher requirements.
According to relevant information, anti-counterfeiting large models have become new opportunities for anti-counterfeiting in the era of large models. Under the influence of the Chain of Thought thinking chain, anti-counterfeiting large models have unprecedented encoding capabilities. They can extend concepts and reason through encoding, fully grasping the internal information contained in the details of the image. At the same time, due to the Scaling Law scale effect, the anti-counterfeiting ability of anti-counterfeiting large models increases linearly with the increase of counterfeit data, and this trend has not yet seen a bottleneck. Anti-counterfeiting large models transform complex problems into data-driven simple problems, avoiding the "carving" thinking of traditional expert models, and greatly improving the anti-counterfeiting effect.
Advertisement
Taking the financial industry as an example, the industry urgently needs a "zero-day repair" plan for "zero-day vulnerabilities" to narrow the risk exposure. MiaoMai Consumption, through its nearly ten years of technological accumulation in the field of artificial intelligence, relies on the advantage of massive basic data and the anti-counterfeiting algorithm library built by real-time updates, providing reference for application scenarios in financial anti-counterfeiting and anti-black production. MiaoMai Consumption's anti-counterfeiting large model uses ultrasonic to establish a cross-modal information mechanism to effectively intercept the pre-attack of deep fakes. The iteration cycle of the anti-counterfeiting large model is shortened from 90 days to 1 day, and the anti-counterfeiting interception rate is increased from 90.0% to more than 99.9%, realizing a new application model of human-computer collaborative financial anti-counterfeiting. In addition, MiaoMai Consumption has also established the largest black and gray production voiceprint library in the industry, with a data scale of tens of millions, becoming an important "infrastructure" for anti-black production.
"Anti-counterfeiting large model" refers to the complex model built using large model technology to address the problem of forgery and counterfeit detection. Different from traditional expert models, anti-counterfeiting large models have a larger number of parameters and a larger scale of training data. In terms of performance, anti-counterfeiting large models usually have high performance, strong out-of-domain ability, and strong interpretability.Deepfakes are causing and will continue to cause widespread social issues.
In the digital economy era, generative artificial intelligence (AIGC) has attracted widespread attention due to its extensive application in the field of content creation. Deepfakes use deep learning algorithms to simulate and forge audio and video, synthesizing false facial videos by exchanging the identity information of the original and target faces or editing the attribute information of the target face. Over more than a decade of development, deep face-swapping technology represented by "Deepfake" has become more intelligent and highly realistic by applying deep learning technology to face-swapping tasks, creating a huge sensation and receiving extensive attention from academia and the industry. At the same time, technological development is often a double-edged sword. Deep face-swapping technology has been illegally used to seek abnormal benefits and has caused serious harm to the economy, politics, society, and other fields, attracting high attention from all parties.
It was rated as the top of the world's top ten risks. At the beginning of this year, the World Economic Forum released the "Global Risks Report 2024," which listed the false information and false information generated by artificial intelligence as the "top ten global risks in the next two years," fearing that it will further deteriorate the already polarized and conflict-prone global situation. At present, the technology of deep face forgery is still in a rapid development stage. Although the authenticity and naturalness of its generation still need to be improved, this kind of deep face forgery technology can also be easily maliciously used by criminals to make pornographic movies, false news, and even be used for fraud and politicians to create political rumors, which poses a great potential threat to national security and social stability.
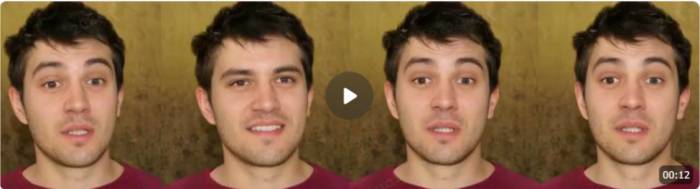
It has been frequently used in the political field abroad. In 2022, deepfake videos about the leaders of Russia and Ukraine were widely circulated on the Internet. In April 2023, the Republican Party in the United States used deepfake technology to release campaign advertisements. In the Democratic Party primaries in January this year, many voters said they received a "call from President Biden" before the primaries, and "Biden" in the call suggested that voters should not participate in the primaries and should save their votes for the November general election for the Democratic Party. This was an attempt by Dean Phillips, a political advisor to Biden's rival, to use "deepfake" to manipulate voters, even bluntly saying: "For only $500, anyone can reproduce my behavior." In March this year, the Indian People's Party released a deepfake video of Rahul Gandhi, the leader of the largest opposition party, the Indian National Congress. In the video, "Gandhi" said a sentence he had never said: "I do nothing." Frequent deepfake videos in India have disturbed the general election. The year 2024 is a "general election year" for the world, with more than 70 countries or regions holding important elections. People are generally worried that artificial intelligence will be weaponized and used to mislead voters, defame candidates, and even incite violence, hatred, and terrorism.
It has lowered the threshold for forgery and fraud. Deepfake technology is often used to impersonate acquaintances or others to commit fraud. In January 2024, criminals successfully forged the image and voice of senior management of a British company using artificial intelligence deepfake technology, impersonating multiple people in online meetings, and defrauding a financial staff member of a Hong Kong multinational company of 200 million Hong Kong dollars. This is not only the most severe "face-changing" case in Hong Kong's history but also the first fraud case involving AI "multiple face-changing." In December 2023, a student studying abroad was "kidnapped," and the parents were demanded a ransom of 5 million yuan by the "kidnappers." According to CCTV reports, in 2022 alone, the United States experienced 2.4 million AI-related fraud cases. In addition, the potential risk of using virtual or synthetic identities to steal or register other people's accounts to defraud pensions and life insurance is also very high.The use of deepfake technology to impersonate official websites or accounts and disseminate false information is not uncommon. In April of this year, Chengqi Assets, a private equity firm with billions of dollars in assets, was impersonated by an individual business operator without private equity qualifications, who registered a WeChat public account called "Private Equity Chengqi," rendering the official account unusable. Similar "Li Gui" incidents also occurred in top private equity firms such as Shifeng Assets and Chengrui Investment. In 2022, two scholars from Stanford, DiResta and Josh Goldstein, investigated and found that more than 1000 LinkedIn accounts were using AI-generated faces as avatars. These fake accounts came from more than 70 companies, pretending to be salespeople sending messages to potential customers, and then connecting interested customers with real salespeople to form an industrial chain.
This has led to a high incidence of financial black and grey industries. Currently, the financial black and grey industries are characterized by high specialization, organization, and chain-like operations, with constantly changing methods and an increasing application of cutting-edge technology, further increasing the difficulty for consumers to guard against them. Lu Quan, Dean of the Artificial Intelligence Research Institute at Consumer Finance, said, "The release of Sora is undoubtedly a significant breakthrough in the field of technology, but it will also lower the threshold for AI forgery, potentially leading to the proliferation of black industry chains such as Deepfake." He also pointed out that multimodal generative large models provide "advanced weapons" for the financial black industry. According to estimates by relevant institutions, in 2023 alone, the economic loss caused by domestic black industry fraud reached 114.9 billion yuan, and the amount of financial business fraud reached 7.5 billion yuan. National regulatory authorities continue to issue early warnings, financial institutions' reputations are severely damaged, and the legitimate rights and interests of financial customers are frequently violated.
The development of deep defense against forgery large models is urgently needed.
(1) Challenges faced by deep anti-counterfeiting detection in the era of large models
Deep forgeries are becoming more realistic. Tang Qianchen from the International Technology Economic Research Institute of the Development Research Center of the State Council pointed out that currently, professional generative AI models such as Midjourney, DALL-E, and Stable Diffusion can generate images that are indistinguishable from reality through user-input images and text. With the widespread application of deep learning technology and AIGC in the field of video and image generation, the quality of current generated videos is increasingly close to real videos and is becoming more widely available at a lower cost. The emergence of large language models for video content generation, represented by ChatGPT 4.0 and DALLĀ·E, has taken the development of face generation to a new level. The continuous release of Sora and ChatGPT-4o undoubtedly proves the possibility of creating more realistic images that understand the physical laws of the real world through large-scale data training of AI models. The mechanism of using diffusion models to train neural networks to reverse the addition of Gaussian noise, that is, synthesizing data from pure noise until a clean sample is produced, makes it difficult for face video anti-counterfeiting detection technology to capture clues of video forgery.The scenario of deepfakes is more complex. Deepfake technology has many applications and risks in typical scenarios such as pornography, fraud, and synthetic speech. Especially with the popularization of open-source code, the ubiquity and universality of this technology are more pronounced, facing the risk of low-threshold diffusion. In recent years, the proliferation of false content has become a disaster, with various forms emerging, such as tampering with elections, spreading fake news, and slandering rumors, showing a trend of popularization, and the application forms are more complex and diverse. Associate Professor Feng Zunlei from Zhejiang University pointed out, "In real scenarios, the anti-counterfeiting detection work of facial videos is easily disturbed by environmental factors. For example, changes in lighting conditions may cause changes in the shadows and highlights of the face, making the face look darker or brighter. Changes in the camera angle may cause the shape and features of the face to deform, making the face look distorted or distorted. In addition, changes in the complexity of the background may also lead to the blurring of the edges of the face or the fusion with the background, making the face look unclear or disproportionate. All of the above factors will affect the authenticity and credibility of the facial video, increasing the difficulty of identification and detection in the anti-counterfeiting detection work of facial videos."
The variants of deepfake technology are faster and more numerous. In the past decade, deepfake technology has become more mature, and after the emergence of generative AI, the diffusion model has solved the problem of posterior distribution alignment of VAEs, the instability of GANs, the large amount of computation of EBMs, and the network constraints of NFs, promoting the continuous increase of the number of deepfakes, and gradually evolving from a single modality to a cross-modal or multi-modality. Wang Lusheng, a researcher at the School of Law of Southeast University, pointed out that deepfake technology is essentially an "unsupervised learning" with strong self-adaptability and rapid evolution, which will make the ability to fake videos leap exponentially. The improvement of capabilities is accompanied by a decrease in costs, and the underground market for attack tools has a considerable scale, usually 200 yuan can buy a targeted attack service. At present, although the anti-counterfeiting effect of anti-counterfeiting detection technology for a single facial video anti-counterfeiting detection dataset is relatively ideal, the generalization performance in cross-dataset anti-counterfeiting experiments still shows obvious deficiencies. At the same time, in real scenarios, due to the unknown methods of facial video forgery, it is difficult to obtain the specific types of forgery methods.
(2) Characteristics of anti-counterfeiting large models
Chain of thought thinking chain, anti-counterfeiting large models have unprecedented encoding capabilities, extending concepts and reasoning through encoding, and fully grasping the internal information contained in the details of the picture. Since its proposal in 2017, the Transformer model has shown unprecedented strength in natural language processing, computer vision, and other fields, and has led to technological breakthroughs such as ChatGPT. The BERT technology based on the transformer has proven to us that all expert questions are essentially encoding problems, and the improvement of encoding capabilities directly affects the accuracy of expert judgment. Research cooperation between scholars from the Shanghai Artificial Intelligence Laboratory and universities such as Beijing University of Aeronautics and Astronautics, Fudan University, the University of Sydney, and the Chinese University of Hong Kong (Shenzhen) shows that MLLMs have demonstrated the ability to proficiently understand the main content of images, and can analyze most of the information in the images based on the proposed queries. In the test of causal reasoning ability for image input, Gemini Pro and GPT-4 can point out many details of the forged face, such as hair, skin, background, etc., without anti-counterfeiting enhancement, like "Sherlock Holmes", which represents a significant improvement in the large model's understanding of the picture.Scaling Law: The anti-counterfeiting capabilities of large models increase linearly with the addition of counterfeit data, and this trend has not yet reached a bottleneck. Researchers Jared Kaplan and Sam McCandlish from Johns Hopkins University have studied the performance of large models in the cross-entropy loss function, indicating that model size, dataset size, and computational volume are negatively correlated with loss. The anti-counterfeiting ability of large models increases linearly with the increase in data and computational volume, and is more efficient. In 2020, OpenAI published a key paper on scaling laws, and in 2022, GPT3.0 demonstrated that ultra-large-scale data can produce the "knowledge emergence" phenomenon. Now, the scale of large models has broken through 100 billion. Anti-counterfeiting large models have the general characteristics of large models, that is, the effective growth of data can promote the simultaneous improvement of model capabilities. With the accumulation and entry of deep fake data, the out-of-domain capabilities of anti-counterfeiting large models are significantly enhanced, which is an improvement of several times compared to traditional expert models.
(III) Anti-counterfeiting large models can transform complex problems into simple data-driven problems.
The academic and industrial communities have proposed traditional expert model detection methods that cover multiple dimensions such as spatial domain, time domain, and frequency domain. These detection methods have achieved certain successes on specific datasets. For example, the typical method based on the spatial domain directly extracts feature information from the spatial domain of video frame images and has performed well in specific types of deep fake detection tasks; the typical method based on the time domain fully utilizes frequency and time domain information and has shown certain advantages in the cross-dataset transfer ability of face fake video detection; the multi-task transfer method uses existing methods in other forensic or visual tasks for transfer and transformation, and applies them to the detection task of face fake videos. Pre-trained on a large-scale lip-reading task dataset, it has shown certain index advantages in both intra-library and cross-library transferability. However, when dealing with the three major challenges of deep fakes, which are "more realistic effects, more complex scenes, and rapid technical variants," traditional expert models usually need to perform complex component disassembly, set different strategies and processing methods for different scenarios. Anti-counterfeiting large models deconstruct this process, establish the large model's cognition of the world's physical knowledge through a large amount of real data, and build the ability to distinguish out-of-domain attacks through a large amount of fake data. On the basis of a fixed model structure, it solves existing and even yet-to-appear complex problems, avoiding the "carving" approach of traditional expert models and achieving a significant improvement in anti-counterfeiting effects.
The technology and application of anti-counterfeiting large models in financial anti-counterfeiting and combating black industry.Deepfake attacks have become a major means of attack faced by the financial industry. The update speed of defense technology has seriously constrained the construction of the financial security defense system. Zero-day vulnerabilities have penetrated from operating systems and computer networks to artificial intelligence, deeply affecting the healthy development of the financial industry. During the time period after the launch of new offensives and before the iteration of defense technology, the operation of institutions is like "naked running". As a leader in the financial industry, Immediate Consumption has introduced anti-counterfeiting large models into daily risk control and anti-fraud management processes, showing the following characteristics:
Short Iteration Cycle
The anti-counterfeiting large model has the characteristic of an unchanging model structure, which can achieve the iteration of fully automated algorithms. When a new deepfake method is announced, the system can automatically generate attack data, evaluate the existing defense capabilities, perform model optimization tasks, test the effects of the iterated model, and release new model inference services, greatly shortening the iteration cycle. The overall process can be reduced from 90 days to 1 day. Immediate Consumption has established a special attack and defense laboratory "Undercover" to track the progress of deepfake attacks and simulate in real time, greatly shortening the discovery cycle of attacks.
Strong Out-of-Domain Performance
Taking the defense effect of "adversarial sample attacks" as an example, the anti-counterfeiting large model can increase the anti-counterfeiting interception rate from 90.0% to more than 99.9%. This is due to the encoding ability of the anti-counterfeiting large model itself, which can cluster attacks that have not been seen in a more "human" way and known attacks in a similar space. Immediate Consumption provides more than 100 types of attack algorithms to generate large-scale fake data, greatly expanding its out-of-domain capabilities.Strong Interpretability
The anti-counterfeiting large model can perform visual attribution analysis for attack data, with the interactive ability to "describe the methods and traces of forgery", greatly enhancing the model's interpretability and user experience, and realizing a new application model of human-computer collaboration in financial anti-counterfeiting. Thanks to the introduction of cross-modal information, during the training process of the anti-counterfeiting large model, a petabyte-level multimodal information is introduced, including a vast amount of text information, voice information, and image information.
In addition to the advantages of a vast amount of basic data and a real-time updated deep forgery algorithm library, Consumption Now also uses ultrasonic to establish a cross-modal information mechanism to effectively intercept the pre-attack of deep forgery. At the same time, Consumption Now has established the largest black and gray industry voiceprint library in the industry, with a data scale reaching tens of millions, creating an "infrastructure" against the black industry.