According to our knowledge, this is the first pure quantum artificial intelligence theory paper published by a Mainland China university team in Nature Communications. Dr. Du Yuxuan from Nanyang Technological University in Singapore said this in reference to his new achievement in collaboration with the team from Wuhan University.
In the paper, he and Professor Luo Yong's research group from Wuhan University described the prediction error and the degree of entanglement, the mathematical theoretical analysis involved, which could be used to study the theory of quantum machine learning.
Many industry insiders believe that quantum computers can bring revolutionary applications and can replace or assist the existing classical computing system in many aspects.
This achievement outlines the theory of quantum artificial intelligence algorithms, which can provide some guidance for the design of all quantum artificial intelligence algorithms.
Firstly, in the fields of chemical materials and biological research, this achievement can help people design more effective quantum artificial intelligence algorithms, which can be used for classifying different molecular structures.It is anticipated that this will greatly facilitate the design and optimization of chemical materials and pharmaceutical molecular structures. For instance, by more accurately classifying and identifying the phase transitions of substances, the development of materials or drugs with superior performance can be accelerated.
Advertisement
Furthermore, this achievement also has significant application prospects for text, language, and image processing.
It can guide people to design more efficient quantum artificial intelligence algorithms, thereby accelerating model training and reducing energy consumption, or achieving better predictive effects with fewer sample sizes.
For example, in the fields of image recognition and natural language processing, quantum artificial intelligence algorithms are expected to significantly improve the accuracy and efficiency of models, thereby enhancing the performance of various intelligent applications.Starting with Feynman and the "No Free Lunch" Theorem
At present, computing power determines the productivity of society. Therefore, the continuous pursuit of high-performance computing devices is one of the core ways for humanity to maintain rapid development.
Classic computing, especially classic computing based on silicon chips, has entered the post-Moore era.
Thus, how to develop new types of computing devices to maintain a steady increase in computing power has become a very concerned issue in academia and the industry.
As one of the highly anticipated architectures for the next generation of computing devices, quantum computing has received tremendous attention.Quantum computers were proposed by Nobel Prize-winning physicist Richard Phillips Feynman, with the core idea of using quantum computers to simulate the behavior of microscopic particles, which can achieve exponential acceleration compared to traditional computers.
After half a century of exploration, it has been found that quantum computers not only have advantages in simulating physical systems but also have many advantages in various computational problems.
For example, in the task of large number factorization, quantum computers can be completed in polynomial time. Traditional computers, on the other hand, require exponential time.
With these advantages, in recent years, academia and industry have been closely monitoring the development of quantum computer hardware and algorithm applications, especially how to fully utilize the advantages of quantum computers by designing efficient algorithms.
The current number of quantum bits in quantum computers, although it has far exceeded the limit of brute force simulation by supercomputers, is still limited due to the inevitable system noise problem, which limits the depth of the circuit and the complexity of the algorithm.Therefore, how to utilize existing quantum devices to implement related applications has become a widely concerned issue in the industry.
Among these potentially implementable applications, quantum artificial intelligence (or quantum machine learning) is considered one of the most promising applications.
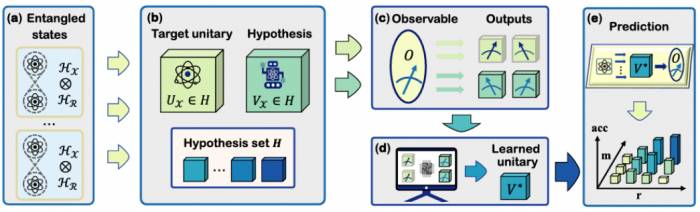
Since 1994, when American computer scientist Peter Williston Shor proposed the quantum large number factorization algorithm, the field of quantum algorithms has ushered in continuous development.
In the field of artificial intelligence, in 2008, a team from the Massachusetts Institute of Technology in the United States proposed the quantum linear solver method, theoretically proving that this method can achieve exponential acceleration compared to classical methods.
In recent years, quantum artificial intelligence algorithms have made a qualitative leap. At the algorithm level, people have started to care about how to couple with existing quantum hardware, that is, how to use existing quantum resources to design algorithms that are adapted to quantum hardware.According to the introduction, the research of this team mainly revolves around the combination of quantum computing and artificial intelligence, divided into two levels:
Firstly, design corresponding quantum artificial intelligence algorithms for different practical tasks, and ensure the feasibility of these algorithms to be executed on existing quantum computers.
Secondly, analyze the upper and lower limits of the capabilities of quantum artificial intelligence algorithms from a theoretical perspective, clarifying in which tasks the relevant algorithms have quantum superiority, such as achieving significant improvements in computation time, energy consumption, and sample size.
This research belongs to the second category of the aforementioned research objectives.
In 2018, IBM first used its quantum cloud platform to realize data classification based on the quantum version of the support vector machine model.In 2021, the team led by Du Yuxuan collaborated with the team of Academician Pan Jianwei from the University of Science and Technology of China, successfully creating a quantum artificial intelligence generation model on a self-developed superconducting quantum computer, and for the first time, generated handwritten digit images using a quantum computer.
The quantum superposition effect and the quantum entanglement effect have long been considered the two core factors determining whether quantum artificial intelligence can surpass classical artificial intelligence algorithms.
Although it is widely believed in the industry that the more quantum entanglement is introduced, the more obvious the superiority of the algorithm. However, in this work, Du Yuxuan and others questioned this view.
Under the framework of one of the core theorems in the field of artificial intelligence, the "No Free Lunch" theorem, they conducted theoretical research.
The so-called "No Free Lunch" theorem refers to the fact that no machine learning algorithm is suitable for all situations. A machine learning algorithm may work well in one field, but may not be effective in another field.Domestic industry dismantles quantum department, research topic once encountered setbacks.
Before the start of this research, there were already 1-2 papers combining quantum machine learning with the "No Free Lunch" theorem in the field, but in Du Yuxuan's view, these results are not perfect.
For example, due to the limited number of observations of quantum states, quantum computing is a probabilistic computing.
However, previous research results were all based on deterministic computing (i.e., assuming an infinite number of observations), which does not conform to the actual situation.This has prompted the research group to deeply contemplate the "No Free Lunch" theorem within the framework of quantum artificial intelligence:
Firstly, under the condition of limited observations of quantum states, how can the "No Free Lunch" theorem be used to characterize the differences in capabilities between classical machine learning and quantum machine learning?
Secondly, how can the "No Free Lunch" theorem be utilized to depict the impact of unique features of quantum computation, such as entanglement, superposition, and coherence, on the capabilities of quantum machine learning models?
As this is a theoretical study, it relies more heavily on mathematical analysis techniques.
At the same time, due to the very short history of research in quantum machine learning theory, the existing mathematical analysis tools in quantum machine learning theory are not sufficient to directly answer the core questions of concern to the research group.For this purpose, they borrowed an interdisciplinary approach from quantum information theory and classical statistical learning theory, integrating the three major fields of machine learning, quantum information theory, and quantum artificial intelligence theory, thereby obtaining relatively satisfactory theoretical results.
When writing the paper, they removed the content that had already been written and was unrelated to the entanglement duality effect.
After the revision, the context of the paper became clearer, and it was able to better demonstrate the diversified impact of quantum entanglement effects on the performance of quantum artificial intelligence models.
This diversified impact is highly related to the number of allowed observations. When there are enough observations, it will have a positive correlation effect on the quantum artificial intelligence model; conversely, it will lead to a degradation of the capabilities of the quantum artificial intelligence model.
During the submission process, in response to the reviewers' comments, the research group stated in their reply: This work is not focused on the general performance study of quantum neural networks, but is based on the principle of Occam's Razor and the "No Free Lunch" theorem (i.e., the two axioms of artificial intelligence), to characterize the impact of entanglement effects on the capabilities of quantum artificial intelligence models.Thus, these results are unrelated to the data and optimizer, hence they possess a deeper and more general nature.
During the period of this study, the domestic industry's layout regarding quantum computing has undergone adjustments, such as dismantling the quantum department or placing greater emphasis on the commercialization and productization of quantum technology, rather than continuing to focus on the exploration of fundamental theories.
"This has, to some extent, increased the difficulty of advancing our work. For example, the first author of our paper had to resign from the intern position and return to school to continue studying this topic," said Du Yuxuan.
Fortunately, the paper was finally successfully published in Nature Communications[1], titled "Transition role of entangled data in quantum machine learning."
Wang Xinbiao, a Ph.D. student at Wuhan University, is the first author, and Professor Tao Dacheng from Nanyang Technological University and Dr. Du Yuxuan, as well as Professor Luo Yong from Wuhan University, serve as co-corresponding authors.Currently, the research team has initiated the next phase of research.
Overall, they plan to enhance the performance and efficiency of quantum computing in applications through more in-depth theoretical research and algorithm optimization.
In terms of theoretical research:
They are exploring whether the "No Free Lunch" theorem can be used to characterize the superiority of quantum computing. At present, a new paper has been published on the preprint website.
In this new paper, they demonstrate that when studying the evolution of quantum states, quantum artificial intelligence algorithms based on quantum memory have a significant advantage in complexity over classical methods.Based on this, they are preparing to generalize the "no free lunch" theorem to more general situations.
The paper published in Nature Communications this time mainly focuses on the theoretical characterization in an ideal environment, but they also hope to study whether the superiority of quantum algorithms in open systems is positively correlated with their internal entanglement degree.
In terms of algorithm design:
Current research shows that when running algorithms on existing quantum cloud platforms, the number of measurements needs to be accurately planned.
Therefore, the next goal of the team's algorithm research is: given a quantum artificial intelligence algorithm, to match its number of measurements and internal entanglement degree in a dynamically efficient way.This ensures that under the existing degree of entanglement, the algorithm will not be limited by the number of measurements, thus being able to fully leverage its advantages.
It is also reported that Du Yuxuan graduated from Sichuan University with a bachelor's degree. He later obtained a master's degree and a doctorate from the University of Sydney in Australia, under the guidance of Professor Tao Dacheng, an academician of the Australian Academy of Science, a fellow of the European Academy, an ACM Fellow, and an IEEE Fellow.
"I am the first student in Professor Tao Dacheng's team to research the direction of quantum artificial intelligence. After completing my doctorate, I joined the JD Explore Research Institute with Teacher Tao in 2021, and I am in charge of the quantum artificial intelligence team," said Du Yuxuan.
"At the same time, I am also a doctoral management trainee at JD, and only less than 50 people can get this opportunity every year," he continued.
In early 2024, due to the difference between personal planning and the company's technological strategic concept, Du Yuxuan chose to leave JD.Currently, he is conducting visiting research at Nanyang Technological University, focusing on the study of quantum artificial intelligence.
To date, Du Yuxuan has published over 30 papers (including 13 first-authored papers).
Through these studies, he was the first to use covering numbers from statistical learning theory to characterize the expressive and generalization capabilities of quantum neural networks, and proposed an automated quantum neural network design framework. In the future, he will continue to delve deeper into the field of quantum artificial intelligence.