Currently, the food delivery business has seen rapid development worldwide, especially in China. With the acceleration of the pace of life, food delivery services have become an indispensable part of daily life.
However, the traditional manual food delivery model faces various challenges and limitations, including restricted delivery distances, complex road environments, low efficiency in closed scenarios such as scenic areas, and delivery obstacles in special situations.
For example, during the outbreak of the COVID-19 pandemic, many places adopted lockdown measures for epidemic prevention.
In view of this, Associate Professor Song Linqi from City University of Hong Kong and his collaborator Meituan began to focus on the unmanned aerial vehicle (UAV) food delivery system.
This system, with its characteristics of speed, low cost, adaptability to various environments and weather conditions, and even adaptability to extreme situations such as epidemic lockdowns, has shown tremendous potential for development.Drone food delivery not only effectively shares the workload of traditional delivery personnel in extreme weather or environments, but also significantly expands the range of users' ordering options, thereby greatly enhancing service efficiency and user experience.
Advertisement
However, in actual operation, the team encountered a key technical challenge: the addresses filled in by users in the food delivery order system are often in various styles, often appearing in an unstructured descriptive manner.
Especially in China, an address may have multiple different expressions, which poses a huge challenge to the rule-based string address parsing solution.
In this context, they decided to take advantage of the rapid development of current natural language processing (NLP, Natural Language Processing) and the large model field, as well as the significant improvement in computing performance and inference speed of graphics processing units (GPU, graphics processing unit), to use artificial intelligence and NLP technology to solve this problem.
In the research, they found that the manually annotated data they used before was a good but relatively expensive method, so they hope to reduce the annotation cost through large models while maintaining the quality of data annotation.However, despite the large models' ability to perform these tasks, they face challenges in the commercial process in the industry, such as legal issues and user data security. In addition, the training and inference costs of large models are high, and they do not have an advantage in speed.
The research group found that large models still have limited capabilities in some specific sub-domains and specific application scenarios, and their performance is not as good as manual data annotation.
Therefore, they chose to use smaller language models for data annotation. This method is not only cost-effective and efficient but also ensures the uniformity of the annotation style.
This is because if the same batch of corpus is annotated by multiple people, due to the different habits and task understanding of each annotator, it is difficult to ensure the consistency of the dataset.
Therefore, although this method may have a gap with manual annotation in accuracy, considering the advantages of cost and efficiency, as well as the uniformity of annotation style, it has great potential for application.In general, this study aims not only to address the address challenges in the drone food delivery system but also to explore new knowledge and methods to promote the widespread application and development of drone food delivery services, thereby bringing more convenient and efficient takeout delivery solutions to society.
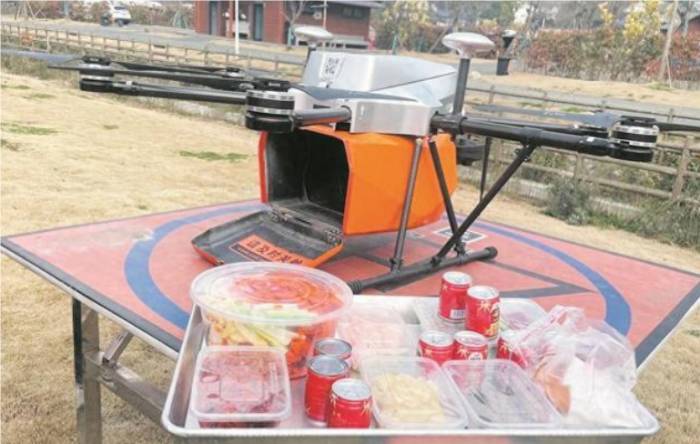
In terms of research details, the research team needs to focus on the feasibility of the technology as well as the needs and limitations of practical applications.
After initial attempts using samples provided by industry partners, they determined to adopt the sequence labeling method in the field of NLP as the main technical approach to solving the problem.
This decision is based on a deep understanding of the nature of the problem and a comprehensive consideration of the feasibility of the technology.
On the basis of the sequence labeling method, they further tried and compared different models, focusing on the key indicators of accuracy and response speed, and ultimately determined the most suitable model scheme.According to the "scaling law" principle in machine learning, the team expanded the scale of the dataset to improve the accuracy of the model.
During this period, they faced the challenges of high data annotation costs and slow iteration speed. Finally, they explored the possibility of alleviating this issue by using some large model products for data annotation.
Song Linqi said: "The success of the project is inseparable from team cooperation and close cooperation with industrial partners. The internship opportunities provided by the cooperative companies allow our researchers to get closer to actual business operations, promoting the implementation of research results."
In the later stages of the project, the research group conducted comprehensive testing and evaluation of the developed system, continuously optimizing the model according to the test results until it met the needs of practical applications.
Through close cooperation and continuous iteration in the above stages, this research not only successfully solved the address recognition problem in the drone delivery system but also explored new methods and ways in the field of data annotation.In fact, at the beginning, the research team was immersed in improving the accuracy of the algorithms. After all, from an academic perspective, precision is one of the key indicators to measure the success of research.
However, when they tried to apply these algorithms to the actual unmanned aerial vehicle (UAV) food delivery system, they faced a significant challenge: the robustness and timeliness of the algorithms.
The engineers from the partner company pointed out to them that although their models performed well on standard test sets, their performance was greatly reduced in the complex and variable actual environment.
This feedback made them realize that there is a certain gap between academic research and practical application, forcing them to rethink and adjust their research direction.
In the process of trying to use large language models for data annotation, another challenge they faced was how to handle and protect the privacy of user data.Due to their data originating from users' historical order records, this involves a large amount of personal privacy information.
Before starting the project, they must conduct a rigorous review and desensitization of the data, a process that is much more complex and time-consuming than anticipated.
Song Linqi said: "The team of the partner company has provided great help and guidance in this regard, and has also made us deeply realize that when dealing with real-world data, it is not just technical issues that need to be considered, but also ethical and legal issues."
Although they have made some progress in data annotation, the partner company quickly pointed out that even large model products cannot be directly applied to the drone food delivery system.
The main limiting factors include processing speed and potential user privacy issues. This made them realize that although large models have outstanding performance in academic research, there are still many practical obstacles to overcome in industrial applications.It is worth mentioning that through this project, they began to shift their focus from pure academic research to conducting research around the actual needs of the industry.
Through close cooperation with partner companies, they not only gained valuable practical experience but also discovered many new research topics and directions.
Recently, the relevant paper was published on arXiv[1] with the title "Can LLM Substitute Human Labeling? A Case Study of Fine-grained Chinese Address Entity Recognition Dataset for UAV Delivery."
Yuxuan Yao is the first author, and Associate Professor Song Linqi from City University of Hong Kong is the corresponding author (who will become a tenured associate professor at the university from July 2024).
In this study, they mainly focused on the use of large models for data annotation in Chinese. Next, on the one hand, they are preparing to train their own models; on the other hand, they will also evaluate the capabilities of existing large models in other aspects.It is also reported that Song Linqi's laboratory mainly engages in the research of artificial intelligence theory and natural language processing, with a particular focus on fine-tuning related to large models, retrieval-enhanced generation, reasoning abilities such as solving mathematical problems, and embodied intelligence.
"We have extensive cooperation with the industry and have sufficient computing power. We welcome students interested in large models to contact us for exchange and cooperation," Song Linqi concluded.