In a recent study, Professor Zhang Yue from Westlake University and his team explored the essential differences between machine-generated text and human-written text, while achieving "fast, accurate, robust, and low cost," clearing the way for the practical application of machine-generated text detection technology.
Specifically, they proposed a new hypothesis for detecting machine-generated text, which led to the creation of a text detection method called Fast-DetectGPT.
They believe that large language models adopt the pattern of empirical risk minimization to learn the collective writing behavior of humans, which has distinct statistical characteristics.
In contrast, human creation is influenced by cognition and intrinsic causality, possessing distinctive individual characteristics.
This results in a significant difference in word choice between humans and machines given a specific context, while the differences among machines are not as pronounced.By leveraging this characteristic, it is possible to use a single set of models and methods to detect text content generated by different source models. At the same time, they use smaller pre-trained language models with fewer than 10 billion parameters to check the text content generated by large language models such as ChatGPT and GPT-4.
Advertisement
That is to say, without the need for training, Fast-DetectGPT can directly use open-source small language models to detect text content generated by various large language models.
Building on the foundation of DetectGPT, the detection speed can be increased by 340 times, and the detection accuracy can be improved by 75%.
In the detection of text generated by ChatGPT and GPT-4, it can even surpass the accuracy of the commercial system GPTZero.
Researchers have indicated that the Fast-DetectGPT algorithm can be easily applied to various pre-trained language models and has good applicability to languages and content from different countries.In the future, Fast-DetectGPT will be applicable to social platforms to distinguish between fake news; it will also be usable on shopping platforms to suppress fake product reviews; and it will be applicable in schools or research institutions to identify machine-generated articles, etc.
By doing so, it can alleviate the potential harm brought about by the widespread use of large language models and help build a trustworthy artificial intelligence system.
"We are also considering deploying Fast-DetectGPT into internet services to provide extensive, real-time detection services," said the researchers.
According to the introduction, the team has been deeply involved in the field of AI for many years. Over the past two years, the rapid development of large language models has profoundly affected people's production and life.
These technologies have greatly improved people's work efficiency in many fields such as news reporting, story writing, and academic research.The widespread use of large language models has also sparked widespread concern and discussion among people about issues such as safety, fairness, and intellectual property rights.
For example, major universities around the world have sparked debates on whether to allow students to use ChatGPT to help complete coursework.
On the one hand, the use of ChatGPT makes it easier for students to get high scores in courses, but it is unfair to other students who have not used such tools.
On the other hand, relying on such tools to complete homework deprives students of the opportunity to exercise and does not achieve the purpose of classroom assignments.
In general, the improper use of some of these technologies has led to the widespread dissemination of false information such as fake news, fake product reviews, and even fake research papers on the Internet, bringing about certain social problems.It can be seen that the content security and reliability issues triggered by large language models have significant social value and are also important topics that urgently need to be studied.
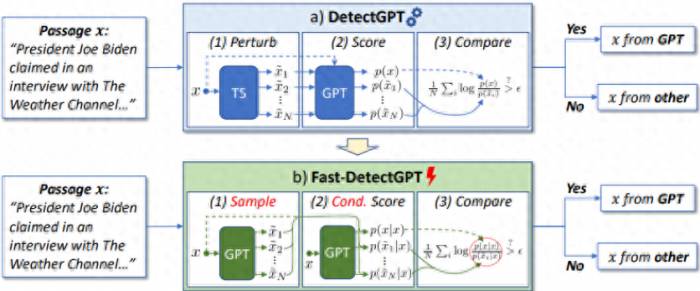
The content generated by large language models is fluent and coherent, even containing many seemingly reasonable specific details and analyses with references.
This makes the content appear particularly credible to ordinary readers, to the extent that they unconsciously accept its content.
However, research has shown that the content generated by large language models, such as ChatGPT/GPT-4, may look serious, but it may contain some fabricated information that is completely inconsistent with the actual situation.
The widespread dissemination of such misinformation on the Internet, on the one hand, will mislead readers to make incorrect cognition and judgment, and on the other hand, it will also damage readers' trust in online platforms, and disrupt the positive interaction between content creators and content consumers on Internet platforms.To build a trustworthy artificial intelligence system, it is necessary to distinguish between content created by humans and content generated by machines. However, this task cannot be accomplished manually.
Firstly, it is difficult for human readers to identify machine-generated content. Research indicates that even linguistic experts struggle to differentiate between text generated by machines and text written by humans.
Secondly, a vast amount of textual content is produced on the internet every day, and manual detection is time-consuming, labor-intensive, and inefficient. Therefore, we need reliable text detection tools to achieve this goal.
A good practical text detection tool should have characteristics such as speed, accuracy, robustness, and low cost, in order to handle the large volume of textual content on the internet.
However, achieving these goals simultaneously presents certain technical challenges.Existing machine-generated text detectors are primarily divided into two categories: supervised classifiers and zero-shot classifiers.
Supervised classifiers are a common type of text detection tool that trains a binary classification detection model by collecting a large amount of machine-generated text and human-written text.
These methods can use pre-trained small models for fine-tuning, thereby achieving rapid detection and low-cost use.
It can cover machine-generated source models, topic domains, and languages on the training dataset, with a high accuracy rate.
However, this method also has a very obvious flaw. Its robustness is relatively poor. When facing generated text from unlearned source models, topic domains, and languages, its performance will be unsatisfactory.Zero-shot classifiers are another type of text detection tool that leverages pre-trained language models to detect machine-generated text.
With extensive corpus learning, pre-trained language models can cover a wide range of topics and languages, possessing good generalization and robustness, and can match the detection accuracy of supervised classifiers.
However, to achieve a better detection accuracy, typical zero-shot classifiers such as the DetectGPT by the team from Stanford University in the United States require about a hundred model calls or a hundred calls to the OpenAI API to complete the detection of a text, which leads to excessive usage costs and longer computation times.
At the same time, DetectGPT relies on the source language model of the generated text for detection, which makes this method unsuitable for detecting text generated by unknown source models.
In recent years, Zhang Yue's laboratory has been focusing on research in trustworthy natural language processing.As researchers in the field of natural neural language technology, while they are creating new technologies, they also truly feel the potential destruction and threats that new technologies may bring.
Preventing these possible damages and threats is their social responsibility as scientific researchers, and establishing a reliable artificial intelligence technology system is their scientific research ideal.
It is understood that the research team did not initially determine whether to conduct machine-generated text detection.
They said: "At the beginning, we were based on such an interest to explore the differences between AI-generated text and human-written text."
So, they analyzed the differences between the two from the perspective of statistical distribution, factuality, and causal relationships.Firstly, they studied the statistical distribution differences between AI-generated text and human-written text, focusing mainly on the characteristics of their distribution in the sampling space.
For this purpose, they analyzed a large number of samples, observing the distribution of each word and the changes in the distribution of preceding and following words.
They found that large language models cannot distinguish between a low-probability event and an incorrect expression in terms of probability distribution. Although the probabilities of both are very small, the difference between the two is huge from a human perspective.
Because one is reasonable content and expression, but it just happens less in reality. The other is an incorrect and incomprehensible expression.
They also found that some very reasonable content written by humans falls outside the sampling space of large language models.These findings all indicate that large language models have a different text generation mechanism from humans, resulting in different text distributions.
Secondly, after determining that there are statistical distribution differences between AI-generated text and human-written text, they believe that this difference can be used to detect machine-generated text.
Combining the extensive social impact of large language models, as well as the research accumulation of the team in trustworthy natural language processing technology, the research group officially established this project.
Subsequently, they reviewed existing machine-generated text detection methods, including trained detection models, zero-shot detection methods, and watermark-based text detection methods.
Then, they chose the zero-shot detection method, conducted a systematic survey of current zero-shot detection methods, and conducted in-depth research on the most powerful zero-shot detection method at the time, DetectGPT.The team found that DetectGPT was extremely slow and had high usage costs, making it difficult to use in practical scenarios.
Combining their understanding of the statistical distribution differences between AI-generated text and human-written text, along with the results and analysis already achieved by DetectGPT and other improved methods, they proposed a new method called Fast-DetectGPT.
The experimental results show that, on the basis of DetectGPT, Fast-DetectGPT can not only improve the detection speed by 340 times, but also increase the detection accuracy by 75%.
Further analysis also revealed that this method can use a fixed small model to detect text content generated by different large models, and the detection accuracy can also be higher than that of DetectGPT.
At the same time, they also found that this method has a close relationship with the commonly used logarithmic probability and entropy baseline, thereby deepening their understanding of the relationship between machine-generated text detection methods.Ultimately, the related paper was published under the title "Fast-DetectGPT: Efficient Zero-Shot Detection of Machine-Generated Text via Conditional Probability Curvature" at the International Conference on Learning Representations 2024 (ICLR 2024).
Xihu University PhD student Guangsheng Bao is the first author, and Professor Yue Zhang from Xihu University is the corresponding author[1].
It is also reported that the detection of machine-generated text is of great significance for building a trustworthy artificial intelligence technology system, and it is an area that the laboratory has been continuously focusing on.
Based on this, they plan to advance subsequent research from two aspects:
On one hand, the current detection of machine-generated text mainly adopts two types of methods: model training and zero-sample statistical testing.These two types of methods for detecting machine-generated text mainly rely on some superficial clues of machine-generated text, such as word usage, grammar, style, and other characteristics, without considering in-depth semantic, knowledge, and pragmatic factors.
With the development of large language models, their ability to simulate human language is also continuously strengthening, making it increasingly difficult to distinguish machine-generated text from surface clues.
Therefore, it is necessary to further combine in-depth semantic information of the text to make more accurate judgments.
On the other hand, the current detection of machine-generated text is still in the stage of empirical research and lacks systematic theoretical guidance.
Thus, it is necessary to delve into some fundamental issues, such as:Where are the limits of machine-generated text detection? What are the fundamental factors that influence it?
Can these fundamental factors be surpassed by large language models, making machine-generated text undetectable?
If so, what capabilities would large language models need to cross this threshold?
By exploring these questions, the team also hopes to make their research findings better contribute to the development of large language models.