Recently, Professor Guo Jingjing's team from the Macau Polytechnic Institute has proposed an AI model named MSSC-BiMamba, which can capture the multimodal data characteristics of polysomnography and enhance the efficiency of sleep staging and sleep disorder diagnosis.
According to the introduction, considering the characteristics of polysomnographic monitoring, the team designed this multimodal sleep state classification model.
The model combines an efficient channel attention mechanism with a bidirectional state-space model, which can effectively capture the multidimensional features and long-distance dependency relationships in the polysomnography data, achieving more accurate sleep staging and sleep disorder classification.
This algorithm has good classification capabilities for the sleep stages of healthy individuals and those with sleep disorders, and it also has high accuracy in the early diagnosis of sleep disorders.
In addition, the algorithm has a parameter volume of only 0.47M, which can achieve rapid inference.This lightweight design allows it to be deployed on various platforms, thereby providing the possibility of cost reduction and efficiency improvement for sleep medicine.
At the same time, this efficient automated sleep analysis system is also expected to become an alternative or supplement to the manual annotation of traditional polysomnography.
Advertisement
It is anticipated that the deep learning sleep analysis algorithm developed in this project will be applied in the following aspects:
Firstly, to serve as an auxiliary diagnostic tool in clinical settings.
The algorithm can be used as a clinical tool to reduce the workload and errors of manual annotation, helping doctors to quickly and accurately analyze patients' sleep data.Secondly, for telemedicine applications.
The algorithm is deployed in the cloud, allowing patients to monitor their sleep at home and upload and analyze data in real time. After remotely reviewing the results, doctors can provide diagnostic advice.
Thirdly, for guiding sleep quality improvement.
Based on the analysis of the algorithm, relevant applications can generate personalized sleep improvement suggestions to help users adjust their lifestyle and sleep habits.
Fourthly, for sleep research.The algorithm utilized in this instance enables the automated analysis of large-scale sleep data, saving time and labor costs and accelerating the progress of sleep science research.
Secondly, it is used for the development of new drugs.
During the process of new drug development, this algorithm can be used to monitor and analyze the impact of drugs on sleep, providing objective data support for the evaluation of drug efficacy.
With the development and popularization of this technology, it is expected that it will not only be used in hospitals and clinics but also integrated into smartwatches and wearable devices, providing real-time sleep monitoring and sleep recommendations.
As is well known, sleep is a fundamental element of human health, involving multiple stages and being crucial for cognitive function and overall health.However, many people are facing various sleep issues, which not only affect the quality of life but may also lead to health problems. Therefore, accurately monitoring and analyzing sleep conditions is crucial for maintaining health.
Currently, monitoring and assessing sleep quality, as well as diagnosing sleep disorders, is a major challenge in the medical field.
Polysomnography is the gold standard for monitoring sleep stages. It includes data such as electroencephalogram (EEG), electrooculogram (EOG), and electrocardiogram (ECG).
Although the data from polysomnography is relatively comprehensive, its analysis process requires specialized equipment and professional physicians for a long time of manual judgment, which is not only time-consuming but also prone to subjective errors.
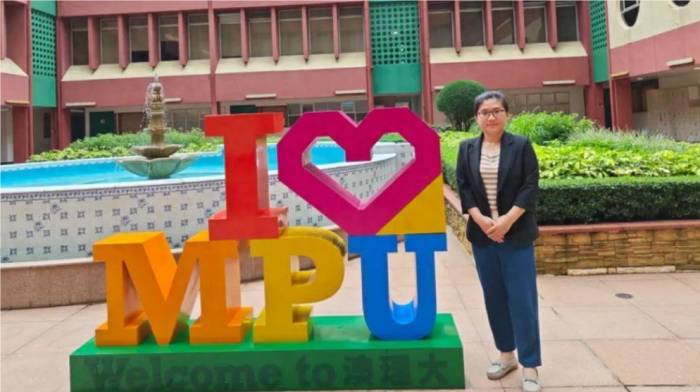
In addition, polysomnography is usually limited to clinical environments and is difficult to popularize into daily life.In fact, this research group has always been focused on the application of artificial intelligence in the field of drug discovery, and this study is their first attempt to venture into the field of digital medicine and smart health.
On a serendipitous occasion, they learned about some hospitals' sleep medicine centers that specialize in the monitoring and diagnosis of sleep disorders.
After communicating with clinical doctors, they deeply felt the tediousness and hard work that doctors experience in the process of manually annotating sleep stages based on polysomnography data.
At that moment, they had an idea: could they develop an AI tool to help doctors free themselves from the heavy annotation tasks?
Later, they realized: given the complexity of polysomnography data, a new type of algorithm must be used to handle it.During a discussion, a team member suggested combining the efficient channel attention mechanism with the bidirectional state-space model.
Researchers stated: "This idea is very innovative because the efficient channel attention mechanism can effectively capture the multidimensional features in the polygraphic sleep data, while the bidirectional state-space model, especially the bidirectional Mamba model, can handle the long-distance dependencies in the data."
Based on this inspiration, they decided to design this multimodal sleep state classification model named MSSC-BiMamba.
It is understood that this is also the first attempt to use the bidirectional Mamba model for multimodal polygraphic sleep data.
Initially, the research group's goal was to classify sleep stages, to distinguish different sleep stages through deep learning algorithms, thereby playing a role in improving sleep quality.During a laboratory discussion, one team member raised such a question: What is the purpose of sleep monitoring? It is to assess whether patients have sleep disorders.
However, most of the current algorithm research is only focused on sleep staging, and there is relatively less research on intelligent diagnosis of sleep disorders. So, can intelligent diagnosis of sleep disorders be conducted in the early stages?
This question ignited the spark of their inspiration, so the research team decided to explore how to use AI algorithms for the diagnosis of sleep disorders.
Subsequently, they selected representative sleep disorder and normal sleep data from public datasets, and used this as the basis to train their deep learning model.
After the data collection was completed, they improved the algorithm and gradually shifted the research focus from pure sleep staging to the more challenging sleep disorder diagnosis.After completing the research, they published the related paper titled "MSSC-BiMamba: Multimodal Sleep Stage Classification and Early Diagnosis of Sleep Disorders with Bidirectional Mamba" on arXiv[1].
Chao Zhang is the first author, and Guo Jingjing is the corresponding author.
Next, the research team will improve the generalization ability and processing efficiency of this model on different datasets, and plan to use it for larger-scale multimodal datasets to verify its effectiveness in practical applications.
At the same time, they will also enrich the data on sleep disorders, such as data on insomnia, sleep apnea syndrome, periodic limb movement disorder, and other diseases.
In addition, the team will further refine the diagnostic criteria to enable the model to more accurately distinguish different types of sleep disorders and provide more targeted recommendations.Finally, they will also develop an online interactive sleep analysis platform, which is expected to allow users to upload sleep monitoring data and receive professional sleep analysis reports and personalized recommendations in real time.