Post-95 Chen Tianle is a doctoral candidate at the University of Maryland, where he completed his undergraduate and master's degrees at Beijing University of Chemical Technology and Johns · Hopkins University in the United States, respectively.
Recently, he and his team have developed a new method based on machine learning neural network algorithms, molecular-scale simulation, and automation technology to discover and optimize biodegradable plastic alternatives.
Moreover, this method can endow materials with programmable optical, thermal, and mechanical properties; at the same time, the material design model has application potential in the fields of tactile sensors, stretchable conductors, electrochemical electrolytes, and thermal insulation aerogels.
This research not only accelerates the process of material development but also significantly improves the predictive ability and optimization accuracy of new materials, creating a new paradigm for the development of new materials and paving a new way for the research and application of environmental protection materials.
The reviewers believe that this research is a "significant advancement in material science research based on repeated experiments."It is reported that in order to advance the application process of all-natural materials, the research team is currently developing product prototypes for common applications such as packaging materials, hoping to conduct preliminary practical application tests in the short term.
Advertisement
Recently, the related paper was published in Nature Nanotechnology with the title "Machine intelligence-accelerated discovery of all-natural plastic substitutes" [1].
University of Maryland doctoral student Tianle Chen, Dr. Zhenqian Pang (now a researcher in the Hundred Talents Program at Zhejiang University), and Dr. Shuai Ming are the co-first authors, Assistant Professor Po-Yen Chen, Professor Liangbing Hu, and Professor Teng Li serve as co-corresponding authors.
Effectively avoid the lengthy process of traditional iterative optimization experiments.The inspiration for this study came from the phenomenon of plastic pollution in the environment. Many years ago, researchers accidentally observed a phenomenon: plastic films floating on the sea surface were mistaken for algae by fish and consumed.
Traditional petroleum-based plastics are difficult to degrade, and natural biodegradable composite materials are one of the ways to solve this problem.
However, traditional material screening methods have problems such as low efficiency and high frequency of experiments, which are difficult to meet the actual application needs, and this study aims to speed up and optimize this process.
Firstly, researchers selected all-natural materials from the Generally Recognized as Safe (GRAS) database, and quickly prepared 286 nano-composite films with different components through automation, and conducted film integrity tests. Subsequently, the data was input into a support vector classifier.
Then, by using active learning algorithms and data augmentation methods to reduce the number of experiments, sufficient training data was provided for the machine learning model, thereby gradually optimizing material performance.After 14 cycles, researchers prepared 135 all-natural nano-composite materials and determined their optical, flame-retardant, and mechanical properties, then constructed an artificial neural network prediction model.
It is worth noting that this prediction model is not only capable of forecasting the physicochemical properties of the constituent materials but also, based on specific user requirements, can automatically design biodegradable plastic substitutes.
Finally, researchers used molecular dynamics simulation to verify and interpret the material performance patterns discovered by machine learning.
Revealing its toughness mechanism from a microscopic scale, after identifying the typical toughening stages in the tensile behavior, the directional preparation of high-performance composite materials was achieved.
The typical toughening stages include: the stretching of hydrogen bonds within the fiber chains to increase modulus, inter-chain slippage to achieve plastic strengthening, and inter-layer crack propagation leading to final fracture.According to the research findings, the integrated workflow from predictive screening to model/mechanism guidance, and then to directed preparation, effectively avoids the lengthy process of traditional iterative optimization experiments, and can promote the multi-attribute optimization of various functional materials.
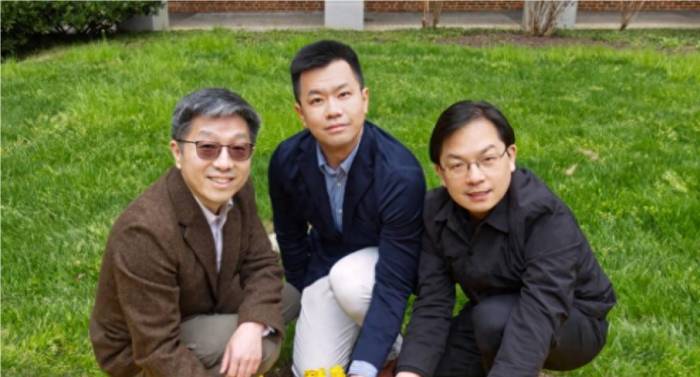
Technical advantages: efficient material screening, accurate prediction, multi-function optimization
At the initial stage of the project, researchers plan to complete the training of the model by collecting data from existing literature to recommend promising materials.
However, as the research progresses, they found that the performance of nanomaterials is easily affected by the batch of raw materials and the preparation process.Chen Tianle explained, "There are significant differences in the selection of raw materials, sample preparation, and data reporting methods among various literatures, which makes it difficult for us to collect highly consistent data for model training."
Based on this, they decided to complete all data collection within the laboratory, using automation technology to achieve high-throughput sample preparation, and reducing the required data points through active learning.
During the research process, the team members noticed that expanding the types of raw materials can significantly broaden the performance range of composite materials.
Therefore, by introducing transfer learning methods and adding chitosan to the material system, the mechanical properties of the material under high strain were significantly improved, extending the application potential of the material.
From the significant improvement in mechanical properties, the researchers prepared composite film materials after post-processing (including calcium ion cross-linking and hot pressing), with a maximum tensile strength of 520MPa.Compared to traditional iterative optimization experiments, this technology has the advantages of high-throughput material screening, precise prediction, and multifunctional optimization.
Specifically, researchers used robotic technology and automated systems to efficiently and quickly prepare and test more than 400 different nano-composite membrane materials.
"Through the automated sample preparation system, not only has the experimental time and manpower input been greatly reduced, but also the consistency and reliability of sample preparation have been improved, which allows us to process and test hundreds of material samples in a short time," said Chen Tianle.
In addition, researchers used active learning to collect data points with information value, reducing the amount of data required to build a machine learning model.
After 14 rounds of active learning cycles, the average relative error of the model was reduced to about 17%, close to the experimental error range of actual measurements. Such high-throughput, high-efficiency experimental design can more quickly carry out material verification and optimization.Traditional methods require a large number of trial-and-error experiments to determine which material combinations can meet specific performance requirements. This process is both time-consuming and costly.
The team utilized a machine learning model based on artificial neural networks to achieve high-precision prediction of various properties of nanocomposite materials.
By building and training the prediction model, it is possible to directly predict the optical, thermal, and mechanical properties of materials, thereby quickly and efficiently determining the target formula.
Unlike traditional single-performance optimization methods, this technology can not only predict the various properties of materials but also perform reverse design according to specific application requirements.
Chen Tianle gave an example, saying that if a material with both high strength and high transparency is needed, the model can screen out the best combination that meets these conditions from a vast material database and provide the corresponding preparation method.He stated: "This ability for reverse design enables us to develop high-performance all-natural materials that meet a variety of application needs in a shorter period of time, more flexibly. Moreover, the composite material system can cover most engineering plastics in terms of mechanical properties."
Committed to establishing a model that includes more materials
The research was jointly completed by three research groups, which discussed the selection of raw materials, the choice of performance indicators, and the actual application plan together.
Among them, Professor Chen Boyan's research group is responsible for the automated material preparation and the construction of the machine learning model, including the rapid preparation of a large number of sample materials using robotic technology, and performance prediction and optimization through machine learning algorithms.Professor Hu Liangbing's team focuses on the preparation of cellulose materials and the post-processing of composite materials, ensuring the reliability of the materials in various performance indicators and conducting necessary experimental verifications.
Professor Li Teng's laboratory conducts molecular dynamics simulations, understanding the micro-behavior and performance mechanisms of materials through theoretical simulations, providing theoretical support for model predictions.
The first author of the paper, Chen Tianle, studied polymer materials and engineering at Beijing University of Chemical Technology. Under the guidance of Professor Liu Jun, he participated in the molecular dynamics simulation research on the stretching process of graphene/styrene-butadiene rubber composite materials.
Later, he obtained a master's degree in chemical and biomolecular engineering at Johns Hopkins University in the United States. During his master's studies, Chen Tianle explored the molecular dynamics and density functional theory calculations of the dynamic structure of nitride films at extremely low temperatures under the guidance of Professor Paulette Clancy.
In 2020, he came to the University of Maryland to pursue a Ph.D. under the guidance of Professor Chen Baiyan. Currently, the main research direction of the team is to discover advanced materials through machine intelligence and robotic automation.While this study has made progress, it is important to understand that although the study can achieve precise modeling, the existing material library is still relatively limited. Therefore, the research team plans to further expand the material library to broaden the performance range of composite materials.
At the same time, researchers are developing material coding methods to support model scalability, so that the model can handle new materials without the need for a large number of re-experiments. In addition, they are exploring various manufacturing and post-processing methods to enhance the water stability of materials.
In this field, one of the major challenges of AI applications is the quantitative general predictive ability. Although there are many large language models that can provide broad summaries based on the analysis of literature data, it is difficult to accurately predict the performance of nanocomposite materials.
Now, the team is working on establishing a model that includes more materials.
Chen Tianle said: "We hope to develop a universal material coding method in the future, which can not only capture the similarities and correlations between different material types, but also express the differences between material variants, and adapt to various scales to enhance the universality and adaptability of the model."
(Note: The name "Chen Tianle" is a direct translation and may not be accurate if it is a proper noun that should be kept in its original form.)