In the deserts of Australia and South Africa, astronomers are deploying a vast array of metal detectors. In the future, these detectors will work simultaneously to search for radio signals in the universe.
Within the next five years, the Square Kilometer Array Observatory (SKA) will officially launch, seeking new information about the first stars in the universe and the various stages of galaxy evolution.
However, after tens of thousands of dish antennas and antennas are fully synchronized, astronomers will soon face a new challenge. They will need to sift through approximately 300 petabytes of cosmic data each year, enough to fill one hundred million laptops.
Over the next decade, this issue will continue to arise in other observatories. As astronomers build more and more giant cameras to image the entire sky and launch infrared telescopes to search for distant planets, the scale of data they collect will be unprecedented.
Cecilia Garraff, a computational astrophysicist at the Harvard-Smithsonian Center for Astrophysics in the United States, said: "We are completely unprepared, and it's time to be anxious. When you have too much data but lack the technology to process it, it's like having no data at all."To tackle the challenge of an overwhelming amount of data, astronomers are turning their attention to artificial intelligence and optimized algorithms to capture hidden patterns in vast and complex datasets.
Advertisement
Some are currently working on establishing research institutes that combine computer science with astronomy, and striving to build new collaborative relationships.
In November 2022, Gaff launched AstroAI as a pilot project at the Harvard-Smithsonian Center for Astrophysics.
Since then, she has assembled an interdisciplinary team of over 50 members, planned dozens of projects, focusing on the earliest appearance of the universe, as well as whether we are the only life within it.
In the past few years, several similar collaborative organizations have followed Gaff's approach and are now competing for funding to expand to large institutions.Galaf recognized the potential of artificial intelligence models throughout her career in astronomy, physics, and computer science. In past collaborations, language barriers have been a major obstacle she faced.
Usually, it is difficult for astronomers and computer scientists to work together because they use different jargon to describe similar concepts. Galaf is no stranger to translation challenges, having grown up in Argentina but attended a school that taught entirely in English, where she had similar experiences.
She draws on this experience, trying to bring people from both communities together so that they can identify common goals and find ways to communicate.
Astronomers have been using artificial intelligence models for many years, primarily for classifying known objects such as supernovae in telescope data.
When the Vera C. Rubin Observatory launches in 2025, this image recognition capability will become increasingly important, as the number of supernova detections will jump from thousands to millions per year.The new wave of artificial intelligence applications goes far beyond the realm of matching games. Recently, algorithms have been optimized to perform "unsupervised clustering," in which they can capture patterns in data without being told specifically what to look for.
This opens the door for astronomers to find effects and relationships they currently do not know about. Gálvez said that these computational tools have provided astronomers with the ability to "systematically search for the unknown" for the first time.
In January 2024, researchers at AstroAI classified over 14,000 detection data from X-ray sources using this method. Without this method, it would have been difficult for them to complete this task.
Another way artificial intelligence plays a role is in detecting the chemical composition of the atmospheres of other planets. Astronomers use telescopes to analyze starlight that passes through the planetary atmosphere and is absorbed by different molecules at specific wavelengths.
To figure out the remaining spectrum, astronomers usually compare it with the fake spectra they generate based on a few molecules of interest, such as water and carbon dioxide.Exoplanet researchers hope to expand their search scope to cover hundreds or even thousands of compounds that may indicate the presence of life on a planet. However, currently, it takes us several weeks to find just four or five compounds.
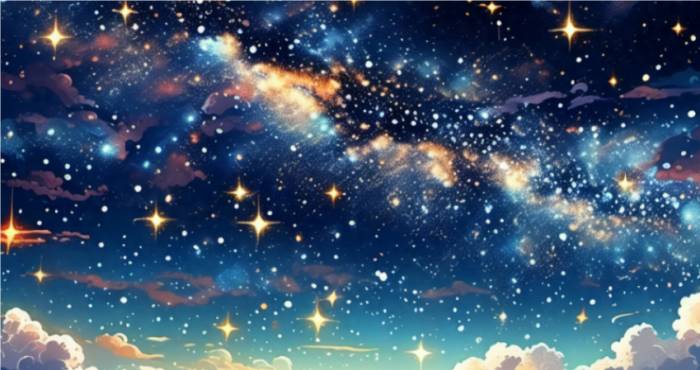
Thanks to the newly deployed James Webb Space Telescope and the Ariel Space Telescope, which the European Space Agency plans to launch in 2029, the number of exoplanets we detect will increase from dozens to thousands, and the efficiency bottleneck in exploring compounds will become increasingly troublesome.
Astronomer Mercedes López Morales, who studies exoplanet atmospheres at the American Center for Astrophysics, said, "Dealing with all these observations was originally an impossible task for us. The emergence of something like AstroAI is just in time, just before this flood of data comes to us."
In 2023, López Morales collaborated with Mayeul Aubin, an undergraduate intern at AstroAI at the time, to establish a machine learning model that can more effectively extract molecular composition from spectral data.
Within two months, their team built a model that can search the spectra of thousands of exoplanets in 31 seconds, looking for the characteristics of five different molecules. This achievement won them the highest award in the European Space Agency's Ariel Data Challenge.Researchers hope to train a model to seek out hundreds of additional molecules, thereby increasing their chances of detecting signs of life on distant planets.
The collaboration with AstroAI has also produced realistic simulations of black holes and maps of the distribution of dark matter throughout the universe.
Galaf's goal is to eventually establish a large language model similar to ChatGPT. This model is trained on astronomical data and can answer questions about observations and parse literature to obtain supporting evidence.
Astronomer and data scientist at the Netherlands Institute for Space Research, Daniela Huppenkothen, said: "It's like having a huge new playground to explore. We can use artificial intelligence to solve problems that were previously unsolvable due to the high computational costs."
However, as Huppenkothen outlined in a recent preprint, incorporating artificial intelligence into the astronomical workflow also has its own trade-offs. Although AI models are efficient, they often operate in ways that scientists cannot fully understand.This opacity makes their debugging complex and also makes it difficult to determine how they introduce bias. Like all generative artificial intelligence, these models are prone to hallucinations, creating non-existent relationships, and finally reporting their conclusions with unwarranted confidence.
Hupeng Kessen said: "It is important to critically examine the role and failure of these models. Otherwise, we will view how the universe operates from a perspective that contradicts the facts."
Researchers are working to incorporate errors into the algorithm's response to explain new uncertainties. Some people believe that these tools can ensure an additional layer of review in the current publication and peer review process.
"As humans, we are naturally inclined to trust machines," said Viviana Acquaviva, an astrophysicist and data scientist at the City University of New York, who recently published a textbook on the application of machine learning in astronomy.
"We need to clearly demonstrate the results that are often not clearly explainable, while being very honest about our capabilities."Researchers have recognized the ethical consequences of introducing artificial intelligence, even in seemingly harmless environments such as astronomy.
For instance, if only specific institutions have access to the computational resources required to run these tools, these new artificial intelligence tools may perpetuate existing inequalities in the field.
Hu Pengkeson said that if astronomers reuse existing artificial intelligence models trained by companies for other purposes, they would "inherit many of the inherent moral and environmental issues within these models."
Galaf is working to address these issues. The AstroAI models are all open-source and free. The organization will also provide assistance to adapt the models to different astronomical applications.
She is also collaborating with the Berkman-Klein Center for Internet & Society at Harvard University to formally train an artificial intelligence ethics team and learn best practices to avoid biases.Scientists are still researching how the advent of artificial intelligence might impact astronomy. If AI models can propose entirely new ideas and provide scientists with new avenues for research, it will forever change the role astronomers play in deciphering the universe.
However, even if artificial intelligence remains merely an optimization tool, it will become a powerful weapon in the arsenal of cosmic exploration.
"Artificial intelligence will change the game," said Jia Laifu, "We can't rely on ourselves alone anymore."