Recently, Assistant Professor Shi Lei from Kennesaw State University in the United States, along with collaborators, has achieved further breakthroughs in the field of cardiac mechanics modeling by introducing a digital twin technology based on an inverse finite element analysis (iFEA) framework, utilizing real-time changing medical image data to estimate the mechanical properties of cardiac tissue.
Through this, they have overcome the limitations of existing cardiac mechanics models in processing dynamic image data.
With the help of the inverse problem method, the research team has deduced new knowledge about the mechanical properties of cardiac tissue, providing more reliable tools for personalized medicine.
The expected potential applications of this achievement include:
Firstly, it can be used for personalized diagnosis and treatment planning of heart diseases.By utilizing individualized cardiac mechanics models, physicians can diagnose heart diseases more accurately, including conditions such as hypertrophic cardiomyopathy and myocardial infarction, and formulate more precise treatment plans for patients.
Advertisement
Secondly, for preoperative simulation and planning.
That is, this model can assist cardiac surgeons in simulating and planning before surgery, providing references for surgical pathways, instrument selection, and surgical plans, thereby enhancing the accuracy and safety of the surgery.
Thirdly, for the development and evaluation of heart disease drugs.
The development and evaluation of drugs for heart diseases greatly benefit from personalized cardiac mechanics models, and these models can be used to assess the effectiveness of drugs, accelerating the development process of new medications.Fourthly, for heart health monitoring and prevention.
This technology can be combined with real-time heart image capture to achieve monitoring and prevention of heart health status.
By continuously tracking the changes in the mechanical properties of heart tissue, potential heart problems can be detected early and preventive measures can be taken.
Fifthly, for virtual surgery and education and training.
A virtual surgery system based on this model can be used for medical education and training, allowing medical students and intern doctors to learn heart surgery techniques in a simulated environment.Section Six: Application in Personalized Management of Heart Disease Patients.
By integrating clinical data with personalized heart models, personalized management and continuous monitoring can be achieved for heart disease patients, thereby providing them with more accurate treatment plans and prognostic assessments.
In summary, this achievement is expected to promote the development of personalized medicine in the field of cardiology, bringing new impetus to the diagnosis, treatment, and prevention of heart disease.
It is reported that the digital twin technology of biological systems and organs can combine detailed digital models of biological systems or organs with the actual biological body.
Using this technology, the functions and states of biological systems can be monitored, simulated, and analyzed in real time, thereby achieving more accurate medical diagnosis, personalized treatment plans, and cutting-edge biomedical research.As a form of digital twin technology, personalized cardiac mechanics modeling in the human body is primarily aimed at creating customized biomechanical modeling based on the anatomical and functional characteristics of an individual's heart.
It combines clinical image data of specific patients with mathematical models to perform precise mechanical analysis and simulation of the patient's heart, thereby achieving personalized understanding and personalized prediction of cardiac function and pathological states.
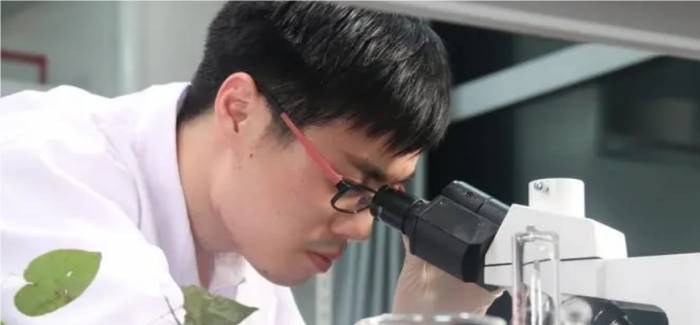
However, for existing models, they can only be used with medical images obtained in a single cardiac phase, which limits their applicability in handling dynamic image acquisition.
Therefore, Shi Lei hopes that this research can address the following issues:
Firstly, to tackle the challenge of dynamic image data processing.The current cardiac mechanics models are primarily based on static image data, which struggle to meet the demands of dynamic image acquisition.
Therefore, he and his collaborators hope to utilize time-series medical image data, employing deep learning methods, to achieve precise estimation of the mechanical properties of cardiac tissues.
Secondly, solving the inverse problem.
A key issue faced by traditional cardiac mechanics modeling is that parameter estimation is usually forward, that is, simulating cardiac behavior from known physical properties.
In contrast, this study adopts the method of inverse problems, inferring the mechanical properties of cardiac tissues from observed data, i.e., from medical images.Once again, establishing a more realistic heart simulation.
By introducing anisotropic hyperelastic constitutive models and physiologically relevant boundary conditions, this study aims to develop a framework that can more realistically and accurately simulate the mechanical behavior of myocardial tissue.
Finally, explore the relationship between optimization algorithms and model parameters.
To explore this issue, the study also involves the selection of optimization algorithms and sensitivity analysis of optimization model parameters, aiming to explore the robustness and effectiveness of the optimal model parameters.
It is understood that Shi Lei has mainly studied topics related to mechanics before, such as aerospace, mechanical structures, and materials.Later, he found that applying the knowledge of mechanics and mathematics he had learned to solve complex biological system problems was truly wonderful.
Previously, when he was pursuing his Ph.D. in the Department of Mechanical Engineering at Columbia University in the United States, he mainly used traditional physical and mathematical tools to construct modeling problems that conform to the characteristics of biological entities.
During his Ph.D., he also met many doctors and various experts in New York.
After graduating with his doctorate, he did not leave but continued to work in the Computational Cardiovascular Laboratory of Assistant Professor Vijay Vedula at Columbia University, starting research in the field of cardiovascular studies.
It was during this period that Shi Lei began this research. Initially, he and Vedula first determined the research objective: that is, to estimate the mechanical properties of cardiac tissue using time-sequenced medical image data.Subsequently, they began to conduct research on the background and current state of the field of cardiac biomechanical modeling, and started to design research methods and theoretical frameworks.
This included selecting appropriate mathematical models, structurally anisotropic hyperelastic constitutive models, and optimization algorithms, among others.
Subsequently, they collaborated with world-renowned cardiac surgery expert Dr. Hiroo Takayama from Columbia University College of Physicians and Surgeons, as well as Assistant Professor Ian Y Chen from Stanford University School of Medicine.
Through this, the research team collected time-series medical image data, including cardiac electron computer tomography (CT, Computed Tomography) image data.
Subsequently, they began steps such as image reconstruction, image segmentation, and image alignment to ensure the quality and consistency of the data.Next, the team began to develop models and develop algorithms. Ultimately, they integrated the algorithm into the open-source software svFSI (), which was jointly developed and maintained with the Stanford University team.
When setting boundary conditions, Shi Lei and others conducted in-depth theoretical calculations and had repeated discussions with doctors, ultimately designing reasonable boundary conditions.
They found that many boundary conditions used in previous famous studies were actually unreasonable and could not truly reflect the state of the heart in the body.
In fact, just the single task of "setting boundary conditions" is enough to become an independent paper.
However, they decided to integrate these works into a comprehensive paper, allowing readers to gain a more comprehensive harvest through a single paper.Ultimately, the relevant paper was published under the title "An Optimization Framework to Personalize Passive Cardiac Mechanics" on arXiv[1].
Subsequently, the research team plans to embark on new explorations in the following areas:
Firstly, integrating deep learning and graph neural network technology.
That is, to explore how to further integrate deep learning and graph neural network technology into the process of solving the inverse problem of cardiac mechanics modeling.
For instance, in the second paper that the researchers are about to publish, they further optimized the inverse finite element analysis framework.This can improve the accuracy and efficiency of parameter estimation through graph neural networks, achieving more precise estimation of the mechanical properties of cardiac tissue.
Using this framework, calculations that previously took days or even weeks to complete can now produce results in just a few seconds, significantly reducing the cost of medical applications.
Secondly, expand to other medical application areas.
Based on the successful application of graph neural networks in solving traditional finite element calculation problems, it is possible to consider expanding this technology to other medical application areas.
For example, it can be attempted to be used in the fields of skeletal biomechanical modeling and brain tissue mechanical analysis, thereby improving the accuracy and efficiency of simulation and calculation in the medical field.Thirdly, develop intelligent models based on graph neural networks.
Intelligent models based on graph neural networks can be further developed to enable rapid modeling and prediction of complex biomechanical systems.
It is expected that these intelligent models will be used to assist in clinical decision-making, personalized treatment planning, etc., promoting the intelligent development of the medical field.
Fourthly, explore interdisciplinary cooperation and application.
In the future, interdisciplinary cooperation with fields such as engineering and computer science can be strengthened to jointly explore the broader application of deep learning and graph neural networks in the medical field.Fifth, carry out clinical practice and application validation.
By collaborating with clinical physicians and researchers, the actual effectiveness and application value of graph neural networks in medical modeling and analysis will be verified, providing more effective support for medical diagnosis and treatment.
It is also reported that regarding his own field of biomechanics, Shi Lei said: "It was established by the famous Chinese scientist Yuan-Zhen Feng and has achieved fruitful results under the efforts of scientists from generation to generation."
With the rapid development of the information age, the complexity of biological bodies has also been explored more deeply, and he believes that this field will usher in significant development.
Therefore, he hopes to attract more talented scholars to join this field, work together to promote human understanding of biological bodies, and strive to solve various disease problems, making more contributions to human health and well-being.